Predictive Modelling for Urban Growth

This Omdena Local Chapter Challenge runs for 7 weeks and is a unique experience to try and grow your skills in a collaborative and safe environment with a diverse mix of people from all over the world.
You will work on solving a local problem, initiated by Nairobi, Kenya Chapter.
The problem
There are several problems related to urban population growth in African cities that can be addressed using machine learning:
- Housing and land use planning: Machine learning algorithms can be used to analyze and predict housing demand, identify optimal land use patterns, and support urban planning decisions.
- Traffic congestion: Machine learning models can be trained to analyze traffic patterns and predict congestion in real-time, helping city planners to optimize traffic flow and reduce congestion.
- Environmental monitoring: Machine learning algorithms can be used to monitor and predict environmental indicators, such as air and water quality, to support sustainable urban development.
- Public services optimization: Machine learning can be used to analyze data on population growth, demographics, and public services usage to optimize resource allocation and improve service delivery in urban areas.
- Predictive maintenance: Machine learning algorithms can be used to predict when public infrastructure such as roads, bridges, and buildings will require maintenance, reducing the costs and disruptions associated with reactive maintenance.
The goals
- Create a housing demand and land use prediction model that can support urban planning decisions and ensure sustainable urban growth.
- Develop a traffic management system that can optimize traffic flow and reduce congestion in urban areas using machine learning algorithms.
- Create an environmental monitoring system that can provide real-time data on air and water quality, and support sustainable urban development.
- Optimize the allocation of resources for public services, such as healthcare and education, using machine learning algorithms that analyze population growth and demographics.
- Reduce the costs and disruptions associated with reactive maintenance by using machine learning algorithms to predict when public infrastructure will require maintenance.
- Develop a tool that can support decision-making by city governments and urban planners by providing insights and predictions based on data analysis.
- Improve the quality of life for residents of African cities by addressing the challenges posed by rapid urbanization, such as housing shortages and traffic congestion.
Why join? The uniqueness of Omdena Local Chapter Challenges
Omdena Local Chapter Challenges are not a competition or hackathon but a real-world project that will grow your experience to a new level.
A unique learning experience with the potential to make an impact through the outcome of the project. You will go through an entire data science project lifecycle. This covers problem scoping, data collection, and preparation, as well as modeling for deployment.
And the best part is that you will join the global and collaborative community of Omdena with tons of benefits to accelerate your career.
First Omdena Local Chapter Challenge?
Beginner-friendly, but also welcomes experts
Education-focused
Open-source
Duration: 4 to 8 weeks
Your Benefits
Address a significant real-world problem with your skills
Build your project portfolio
Access paid projects (as an Omdena Top Talent)
Get hired at top organizations
Requirements
Good English
Suitable for AI/ Data Science beginners but also more senior collaborators
Learning mindset
This challenge is hosted with our friends at
Application Form



Become an Omdena Collaborator
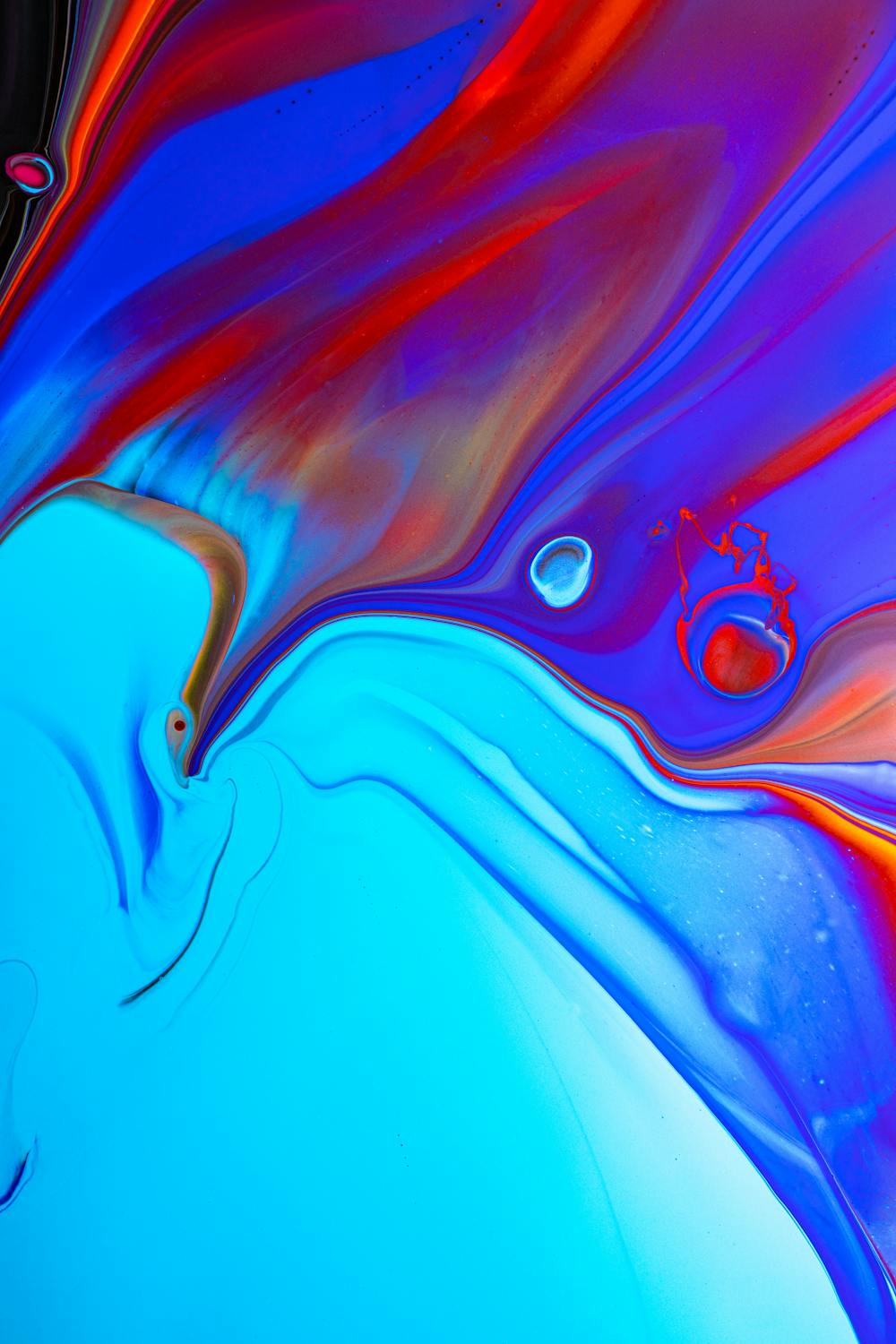