Monitoring Plants Health with AI and Computer Vision

This is a paid opportunity. In order to be eligible to apply for this project, you need to be part of the Omdena community and have finished at least one AI Innovation Challenge.
You can find our upcoming AI Innovation Challenges at https://omdena.com/projects.
The problem
The current approach to plant health assessments and mapping, primarily performed manually by field agents, is labor-intensive and susceptible to inaccuracies. This manual method of monitoring not only requires significant human effort but also introduces the potential for human error, which can affect the reliability of the data collected. Such inaccuracies in assessing plant health, growth stages, and identifying potential issues can lead to suboptimal care and management of plants in nurseries and parklands.
Impact of the Problem:
- Resource Inefficiency: Manual monitoring demands substantial time and labor from field agents, diverting resources that could be more effectively utilized in other areas of plant care and maintenance.
- Reduced Accuracy: Human assessments are prone to subjective judgments and errors, leading to inconsistencies in data on plant health and growth stages. This can result in either over-treatment or under-treatment of plants, both of which can be detrimental to plant health.
- Delayed Response: The time-consuming nature of manual assessments can cause delays in responding to issues like disease outbreaks or nutrient deficiencies. Quick response times are crucial in preventing the spread of diseases and addressing health issues before they cause significant damage.
- Limited Scalability: Manual processes do not scale efficiently as the size of nurseries and parklands increases. The greater the area that needs to be monitored, the more labor is required, making it challenging to maintain consistent monitoring standards across larger spaces.
- Economic Impact: Inefficiencies and inaccuracies in plant health monitoring can lead to economic losses, particularly in commercial nurseries where the health of plants directly impacts revenue. Poor plant health management can result in lower-quality plants that are less marketable or lead to crop losses, directly affecting profitability.
This project aims to address these challenges by digitizing and automating the monitoring of seedling growth and plant health assessments in nurseries and parklands using AI and computer vision technologies. The goal is to increase the accuracy and efficiency of these processes, thereby improving plant care, optimizing resource use, and enhancing the overall management of plant health. By implementing this technology, the project seeks to reduce the reliance on manual labor, standardize data collection, enable real-time monitoring, and provide actionable insights that can be used to promptly address any issues detected, ultimately supporting healthier plant populations and more sustainable park and nursery management practices.
The project goals
The primary goal of this project is to automate the monitoring of seedling growth in nurseries and parklands using AI, particularly through computer vision technologies, to assess plant health, growth stages, and potential issues. This initiative will unfold over a series of planned phases, aimed at enhancing the management and care of plant life within these controlled environments:
- Data Collection and Preprocessing: The first phase involves the collection and preprocessing of images captured by field agents. These images will serve as the foundational data for training machine learning models, focusing on capturing diverse representations of seedling growth stages and health conditions.
- AI Model Development: During this phase, machine learning models will be developed to process the collected images, allowing for the classification of seedling growth stages and health indicators.
- Model Optimization and Integration: The models will be further refined to ensure high classification accuracy, targeted at over 90%.
- Testing and Deployment: Once developed, the models will undergo extensive testing and validation within a test Android application to demonstrate their ability to accurately classify seedlings. This testing will ensure that the model performs reliably under various conditions and accurately reflects the health and growth stages of seedlings.
- System Evaluation and Iterative Improvement: Following deployment, the project will focus on continuously evaluating the system’s performance and making necessary adjustments. This includes refining the AI algorithms based on user feedback and adapting to changes in seedling conditions or environmental factors, ensuring the models remain accurate and effective over time.
- Offline Functionality and Finalization: Design the solution for offline operation to minimize its dependence on network connectivity, enabling its use in remote or low-connectivity environments. The final phase will also involve preparing the initial draft of the Testing and Validation Report and conducting a final project review with stakeholders.
Thus, this project aims to deliver an advanced AI-driven solution that significantly improves the monitoring and management of seedlings in nurseries and parklands. By leveraging cutting-edge computer vision technology and AI, this initiative is expected to transform the care and management practices, leading to more efficient operations, reduced labor costs, and enhanced plant health. This strategic approach promises substantial benefits in nursery and parkland management, contributing to more informed and sustainable practices.
**More details will be shared with the designated team.
Why join? The uniqueness of Omdena Top Talent Projects
Top Talent opportunities come as a natural next step after participating in Omdena’s AI Innovation Challenges.
Everyone in the community is eligible to participate once they have shown the relevant skills based on the merit of involvement in past Omdena challenges and the community.
If you are looking for the next challenge after participating in one or more Omdena AI Innovation Challenges, then we believe you have made the right choice! With a healthy, pressured environment, you will be pushed to contribute, learn and grow even more.
Find more information on how an Omdena Top Talent Program works
First Omdena Project?
Join the Omdena community to make a real-world impact and develop your career
Build a global network and get mentoring support
Earn money through paid gigs and access many more opportunities
Eligibility to join an Omdena Top Talent project
Finished at least one AI Innovation Challenge
Received a recommendation from the Omdena Core Team Member/ Project Owner (PO) is a plus
Skill requirements
Good English
Machine Learning Engineer
Experience working with Machine Learning Computer Vision and/or Data Analysis is a plus.
This challenge is hosted with our friends at
Application Form



Become an Omdena Collaborator
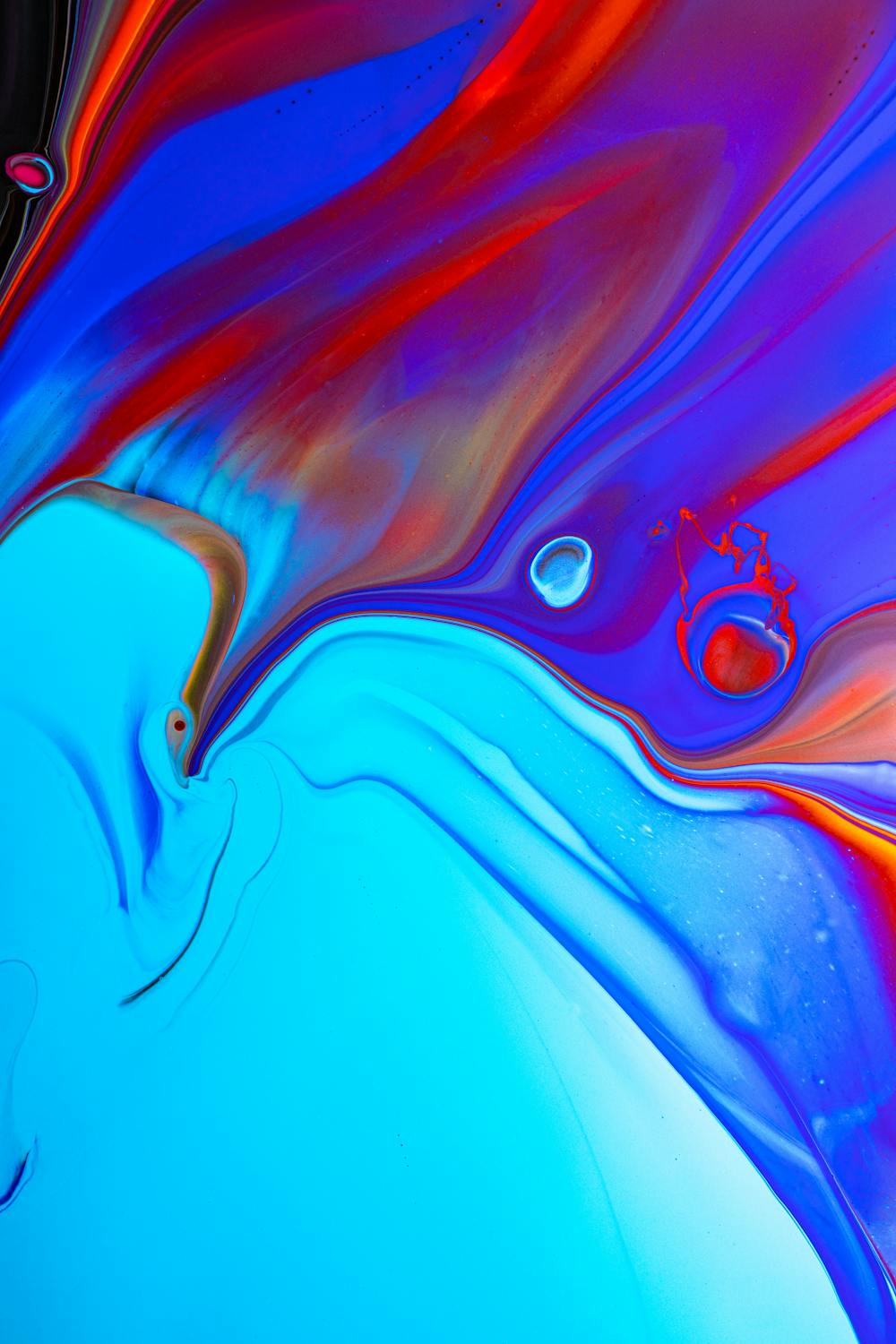