Advanced Crop Image Segmentation with Drone Data for a Mobile Application

Background
DroneAg, an England-based startup, offers a mobile application, Skippy Scout, that automates drone flights to capture crop images, providing actionable insights for farmers and agronomists. A core challenge was optimizing image segmentation to analyze drone-captured imagery and measure crop parameters such as health, type, and area coverage.
Objective
The project aimed to develop a robust segmentation machine learning model capable of extracting meaningful insights about crops, enabling precise monitoring and analysis across multiple drone flights.
Approach
Over an eight-week collaboration, the team employed both unsupervised and supervised segmentation models:
- Unsupervised Segmentation:
- Developed a model yielding satisfactory results with scope for improvement through refined hyperparameter selection and targeted evaluation methods.
- Implemented a pipeline to integrate the model into production workflows.
- Supervised Segmentation:
- Annotated and pre-processed a curated sample of crop images tailored to model requirements.
- Tested multiple supervised models, documenting top performers with recommendations for boosting performance and accuracy.
Results and Impact
The project successfully delivered:
- A machine learning-based crop segmentation pipeline ready for production integration.
- Insights into supervised and unsupervised approaches, paving the way for enhanced crop health monitoring and precision farming.
- Real-world application in Skippy Scout, empowering farmers to optimize their agricultural practices through actionable crop insights.
By improving segmentation accuracy, DroneAg enables more effective crop management, directly benefiting agronomists and farmers in decision-making.
Future Implications
The findings hold significant promise for precision agriculture, including:
- Enhanced policies around sustainable farming using detailed crop analytics.
- Further innovations in drone-based image segmentation for agricultural insights.
- Continued research to refine supervised learning techniques for better scalability and accuracy in diverse farming conditions.
This project underscores the potential of crop image segmentation to revolutionize modern agriculture, fostering smarter, data-driven solutions for global food security.
This challenge has been hosted with our friends at



Become an Omdena Collaborator
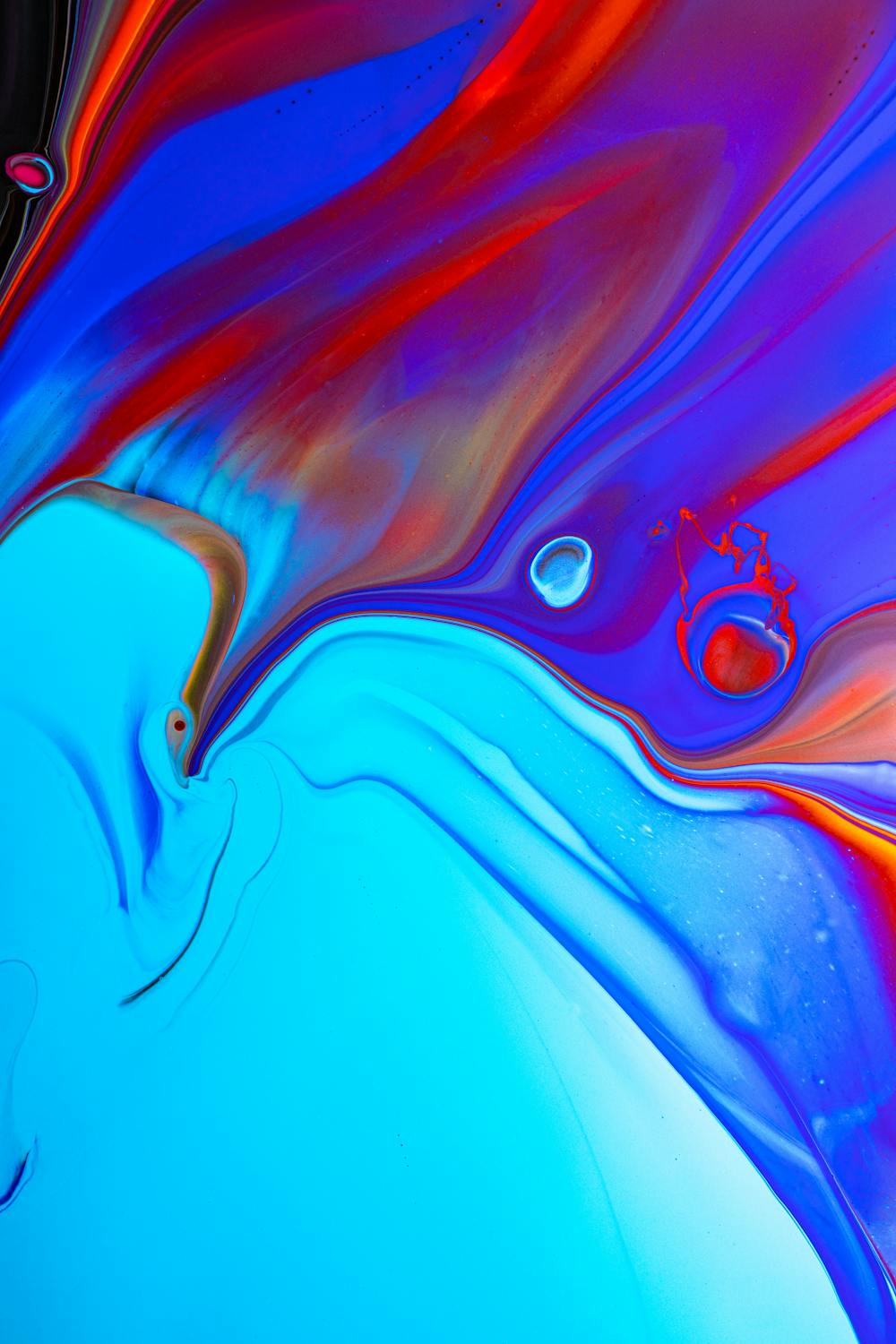