Accurately Identifying Crop Types Using Remote Sensing and Machine Learning

This is a paid opportunity. In order to be eligible to apply for this project, you need to be part of the Omdena community and have finished at least one AI Innovation Challenge.
You can find our upcoming AI Innovation Challenges at https://omdena.com/projects.
The problem
Accurately identifying and mapping specific crop types across large agricultural areas poses significant challenges for farmers, agricultural consultants, and regional planners. Traditional methods of crop identification often involve physical field surveys and manual observations, which are time-consuming, labor-intensive, and can be impractical over larger expanses. Moreover, these methods may not provide the timely data needed for effective agricultural management, such as optimizing resource allocation, monitoring crop health, and planning harvests.
Impact of the Problem:
- Resource Management: Inefficient resource usage, including water, fertilizers, and pesticides, occurs when crop types are not accurately identified and mapped. Precise knowledge of crop distribution allows for targeted application of resources, reducing waste and minimizing environmental impact.
- Agricultural Productivity: Without accurate crop identification, it’s challenging to implement specific agronomic practices tailored to each crop type, potentially leading to suboptimal yields. Farmers may miss the optimal planting and harvesting times, affecting overall productivity.
- Economic Impact: Incorrect or outdated information about crop types can lead to poor decision-making. For example, farmers and investors might make ill-informed choices about crop rotation and land use strategies, impacting profitability.
- Environmental Conservation: Mismanagement of crops can lead to environmental degradation. Overuse of water and chemicals, driven by inaccurate crop data, can lead to soil degradation, pollution, and biodiversity loss, exacerbating the ecological footprint of agricultural practices.
- Policy and Planning: For government agencies and NGOs, inaccurate crop data can hinder effective policy-making and the deployment of agricultural support programs, affecting food security and economic development at a regional or national level.
The goal of this project is to use remote sensing technology and machine learning to accurately identify specific crop types—lettuce, melons, and tomatoes—within a 100 to 500-square-kilometer area. Utilizing high-resolution satellite imagery and advanced analytical algorithms, the project aims to provide precise, up-to-date maps of crop distribution. This information will help optimize agricultural management, enhance resource allocation, and improve strategic planning for crop production and environmental conservation. By implementing this technology, the project seeks to transform traditional agricultural practices into more efficient, sustainable, and productive operations.
The project goals
The primary goal of this project is to accurately identify specific crop types—lettuce, melons, and tomatoes—within a designated area using AI and remote sensing technologies. This initiative will unfold over a series of planned phases, aimed at enhancing agricultural management and precision farming over a 100 to 500-square-kilometer zone:
- Data Collection and Preprocessing: The first phase involves gathering high-resolution satellite imagery and open datasets that include geographical and agricultural data specific to the targeted crops. Information from provided farm locations and detailed insights about tomato and melon farms will be used as reference points.
- AI Model Development: In this phase, models will be developed to identify melons and tomatoes, each with a targeted accuracy of more than 85%. These models will leverage machine learning algorithms to process and analyze the data, distinguishing crop types based on relevant features identified during the data preprocessing stage.
- Testing and Validation: Following the development, these models will undergo rigorous testing and validation processes using real-world data to ensure they meet the accuracy standards set forth. This phase ensures the models’ effectiveness in practical scenarios and assesses their reliability across different conditions and variances in crop appearance.
- Integration and Deployment: The validated models will then be used to create a dashboard to showcase the solution to stakeholders. This deployment aims to provide the stakeholders with actionable insights, enhancing decision-making and operational efficiency.
Thus, this project aims to deliver advanced AI-driven solutions that significantly improve the identification and management of crop types in large agricultural zones. The main objective is to provide substantial benefits in agricultural productivity and resource management, contributing to more informed and efficient agricultural practices.
**More details will be shared with the designated team.
Why join? The uniqueness of Omdena Top Talent Projects
Top Talent opportunities come as a natural next step after participating in Omdena’s AI Innovation Challenges.
Everyone in the community is eligible to participate once they have shown the relevant skills based on the merit of involvement in past Omdena challenges and the community.
If you are looking for the next challenge after participating in one or more Omdena AI Innovation Challenges, then we believe you have made the right choice! With a healthy, pressured environment, you will be pushed to contribute, learn and grow even more.
Find more information on how an Omdena Top Talent Program works
First Omdena Project?
Join the Omdena community to make a real-world impact and develop your career
Build a global network and get mentoring support
Earn money through paid gigs and access many more opportunities
Eligibility to join an Omdena Top Talent project
Finished at least one AI Innovation Challenge
Received a recommendation from the Omdena Core Team Member/ Project Owner (PO) is a plus
Skill requirements
Good English
Machine Learning Engineer
Experience working with Machine Learning, Computer Vision, Remote Sensing and/or Satellite Imagery is a plus.
This challenge is hosted with our friends at
Application Form



Become an Omdena Collaborator
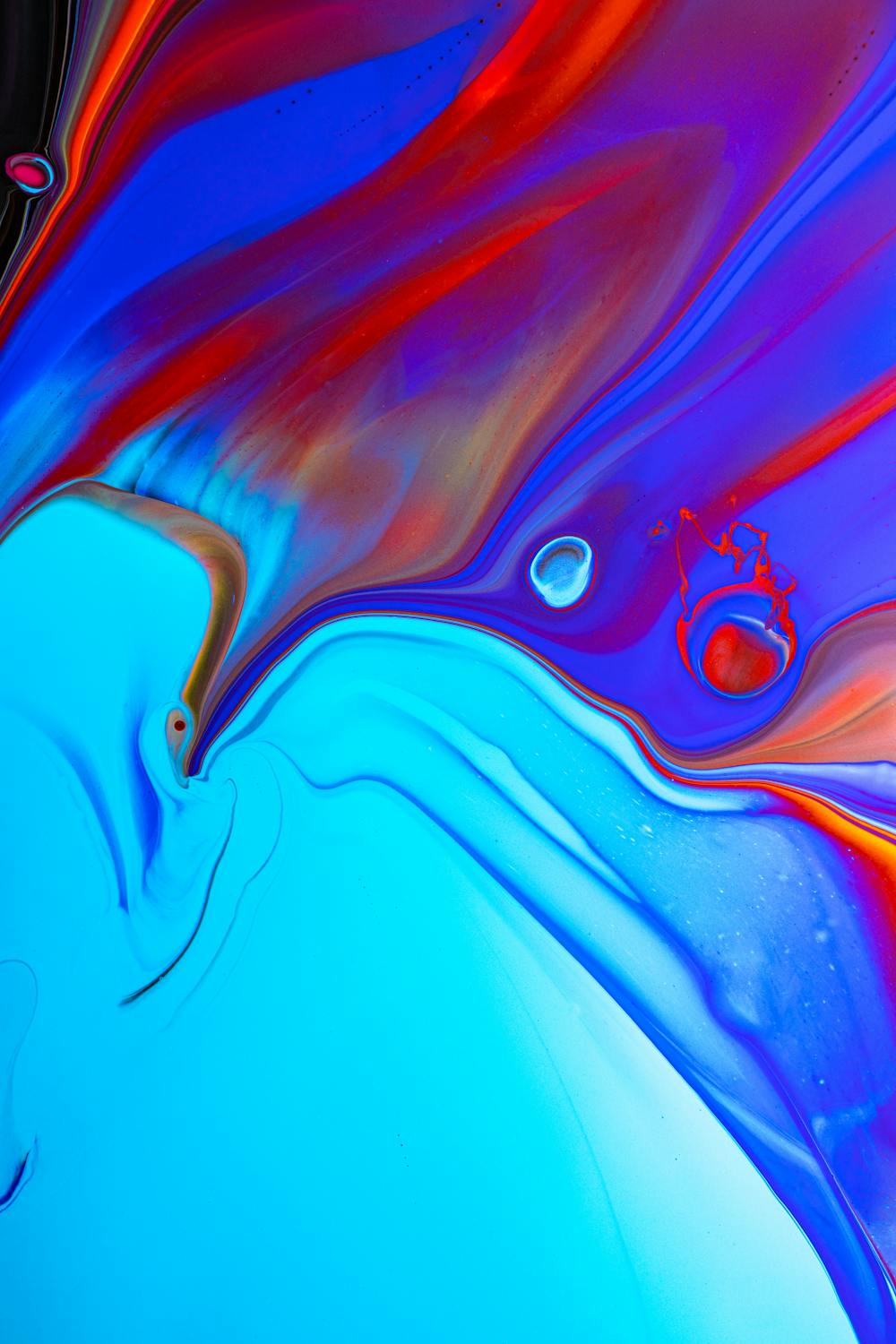