Enhancing Global Mapping Through AI

This is a paid opportunity. In order to be eligible to apply for this project, you need to be part of the Omdena community and have finished at least one AI Innovation Challenge.
You can find our upcoming AI Innovation Challenges at https://omdena.com/projects.
The problem
Global mapping, particularly the accurate mapping and segmentation of buildings, is crucial for a variety of applications, from urban planning and disaster management to service delivery and infrastructure development. However, traditional mapping methods often struggle with issues such as scale, timeliness, and accuracy, especially in rapidly changing environments. The challenge becomes even more pronounced in regions with complex geographic features or dense urban areas where buildings can be closely packed or obscured.
Impact of the Problem:
- Urban Planning and Development: Inaccuracies in building mapping can lead to suboptimal urban planning decisions, impacting everything from traffic management to disaster readiness. Planners rely on precise maps to design infrastructure that meets the needs of growing populations.
- Emergency Response and Disaster Management: In the event of natural disasters, accurate maps are vital for effective emergency response and recovery efforts. Incorrect or outdated building data can hinder rescue operations, delay aid delivery, and complicate recovery efforts.
- Resource Allocation: Governments and organizations use building maps to plan and distribute resources, including utilities and services. Inaccuracies can lead to inefficiencies and inequities in service provision, affecting quality of life and economic opportunities.
- Environmental and Conservation Efforts: Mapping is also essential for environmental monitoring and conservation planning. Precise segmentation of buildings helps in assessing human impact on natural landscapes and planning for sustainable development.
The goal of this project is to enhance the accuracy of global mapping through the fine-tuning of the Segment Anything Model (SAM) with regional weights. This approach aims to improve the model’s performance in identifying and segmenting buildings, leveraging a diverse dataset provided by a partner. The project will explore different methodologies to optimize the SAM model’s accuracy and efficiency, responding to the unique challenges presented by varied regional landscapes and urban configurations. By advancing the capabilities of the SAM model, this project intends to provide more accurate, scalable, and timely building maps that can significantly benefit urban planning, emergency response, resource distribution, and environmental conservation efforts globally.
The project goals
The primary goal of this project is to enhance the accuracy of global mapping and segmentation of buildings using the Segment Anything Model (SAM) with Regional Weights. This initiative will utilize a diverse dataset provided by the partner to refine SAM’s capabilities, making it more effective in identifying and mapping structures accurately across different regions. The project will unfold over the following planned phases:
- Data Collection and Integration: The first phase involves leveraging the diverse dataset provided by the partner. This dataset will be critical in creating a detailed understanding of regional variations in building appearances and layouts, which is essential for the fine-tuning of the SAM model.
- Model Enhancement and Exploration of Approaches: In this phase, the team will focus on enhancing the existing SAM model by integrating regional weights that reflect the unique characteristics of different areas. This involves exploring various approaches to machine learning and image processing to improve the model’s performance in accurately segmenting and mapping buildings.
- Algorithm Refinement: Following the initial enhancements, the project will refine the algorithms based on the performance feedback and additional insights gained during testing. This step is crucial for optimizing the accuracy and efficiency of the model under diverse environmental conditions.
- Validation and Testing: The refined model will undergo rigorous testing to validate its accuracy and reliability. This phase ensures that the model performs well across different regions and can be trusted for use in critical applications such as urban planning and disaster management.
- Documentation and Knowledge Sharing: Ensuring that the improvements and findings from the project are well-documented and shared is vital for future replication and enhancement. This phase will focus on creating comprehensive documentation of the methodologies, algorithms, and results.
Thus, this project aims to deliver a significantly improved SAM model that not only enhances the accuracy of building mapping and segmentation but also supports global efforts in urban planning, environmental conservation, and disaster responsiveness. By providing a more precise, data-driven view of built environments, this initiative is expected to drive better decision-making and resource allocation, thereby contributing to more sustainable and resilient urban and rural development.
**More details will be shared with the designated team.
Why join? The uniqueness of Omdena Top Talent Projects
Top Talent opportunities come as a natural next step after participating in Omdena’s AI Innovation Challenges.
Everyone in the community is eligible to participate once they have shown the relevant skills based on the merit of involvement in past Omdena challenges and the community.
If you are looking for the next challenge after participating in one or more Omdena AI Innovation Challenges, then we believe you have made the right choice! With a healthy, pressured environment, you will be pushed to contribute, learn and grow even more.
Find more information on how an Omdena Top Talent Program works
First Omdena Project?
Join the Omdena community to make a real-world impact and develop your career
Build a global network and get mentoring support
Earn money through paid gigs and access many more opportunities
Eligibility to join an Omdena Top Talent project
Finished at least one AI Innovation Challenge
Received a recommendation from the Omdena Core Team Member/ Project Owner (PO) is a plus
Skill requirements
Good English
Machine Learning Engineer
Experience working with Machine Learning, and/or Computer Vision is a plus.
This challenge is hosted with our friends at
Application Form



Become an Omdena Collaborator
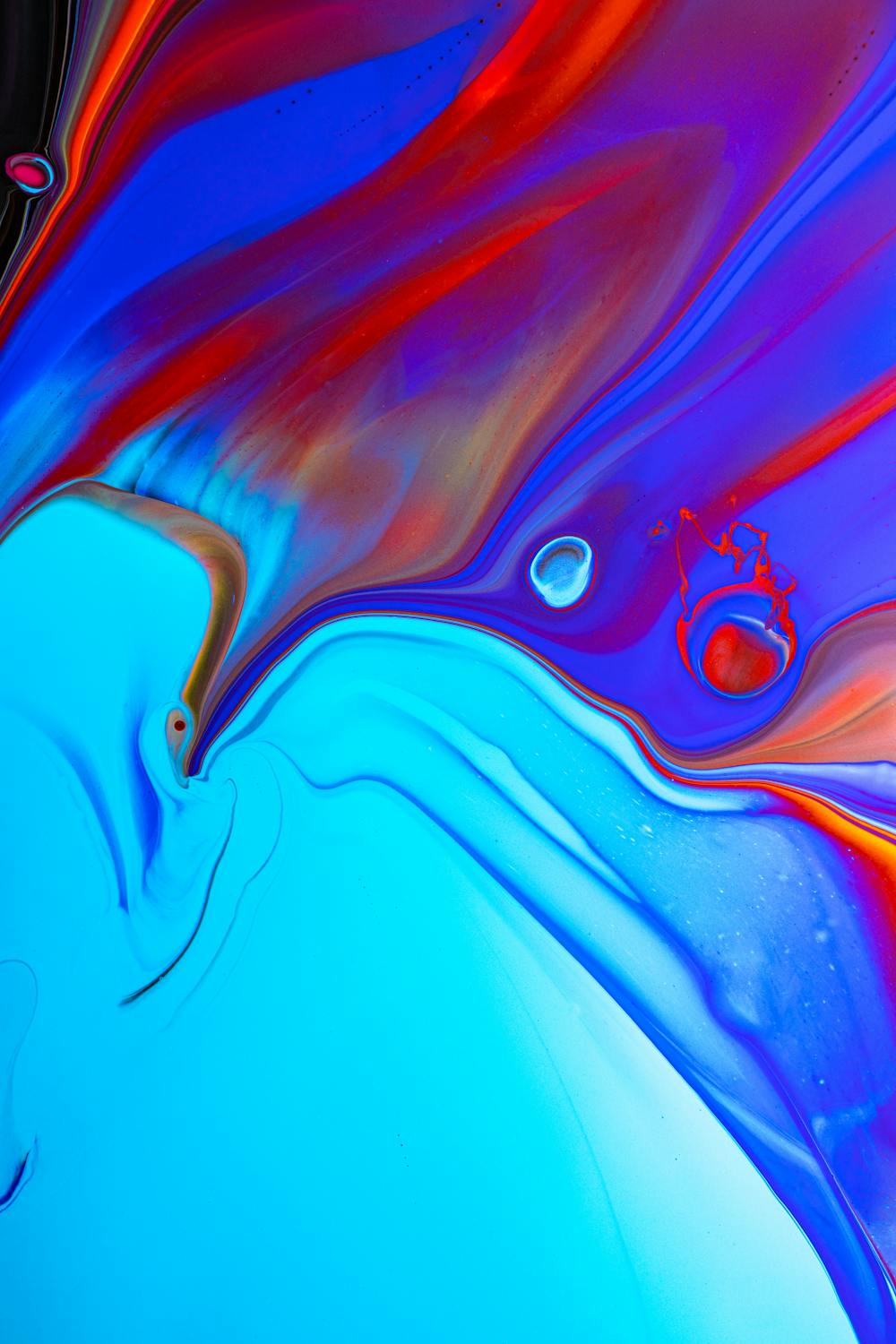