Detecting Data Center Anomaly With Machine Learning and Root Cause Analysis

Background
Data centers are critical infrastructures where anomalies can lead to significant outages. Once an anomaly occurs, Site Reliability Engineers (SREs) face the challenge of manually identifying the root cause by analyzing the sequence of events. This process is time-consuming and labor-intensive. To address this issue, Sensai, an Israel-based startup, partnered with Omdena to automate anomaly detection and root cause analysis, integrating advanced machine learning techniques.
Objective
The primary objective was to automate the Root Cause Analysis (RCA) process for data center anomalies, reducing manual effort, enabling real-time detection, and paving the way for self-healing capabilities in data centers.
Approach
A team of 50 AI engineers collaborated to design a machine learning-based solution that utilized:
- Data Sources: Multivariate time series data collected from machines and applications in hybrid data centers.
- Methods: Applied unsupervised machine learning models and causal inference mechanisms to identify and analyze the sequence of events leading to anomalies.
- Tools and Techniques: Advanced algorithms for real-time anomaly detection and RCA to pinpoint the “most likely” events causing disruptions.
These methods were integrated into Sensai’s data management system to create a robust, scalable automation process.
Results and Impact
The project resulted in:
- Development of ML models capable of real-time anomaly detection and RCA in hybrid data centers.
- Efficient identification of root causes, reducing manual effort and response time for SREs.
- Enhanced system reliability and a step forward toward achieving self-healing data center capabilities.
This project showcases the potential of combining machine learning and causal inference to revolutionize operational efficiency and minimize downtime in data centers.
Future Implications
The findings highlight the transformative potential of integrating causal inference and machine learning in data center management. This approach could:
- Influence industry-wide adoption of automated RCA processes.
- Drive further research into self-healing mechanisms for complex systems.
- Lay the groundwork for more resilient and efficient data infrastructure in the future.
This challenge has been hosted with our friends at



Become an Omdena Collaborator
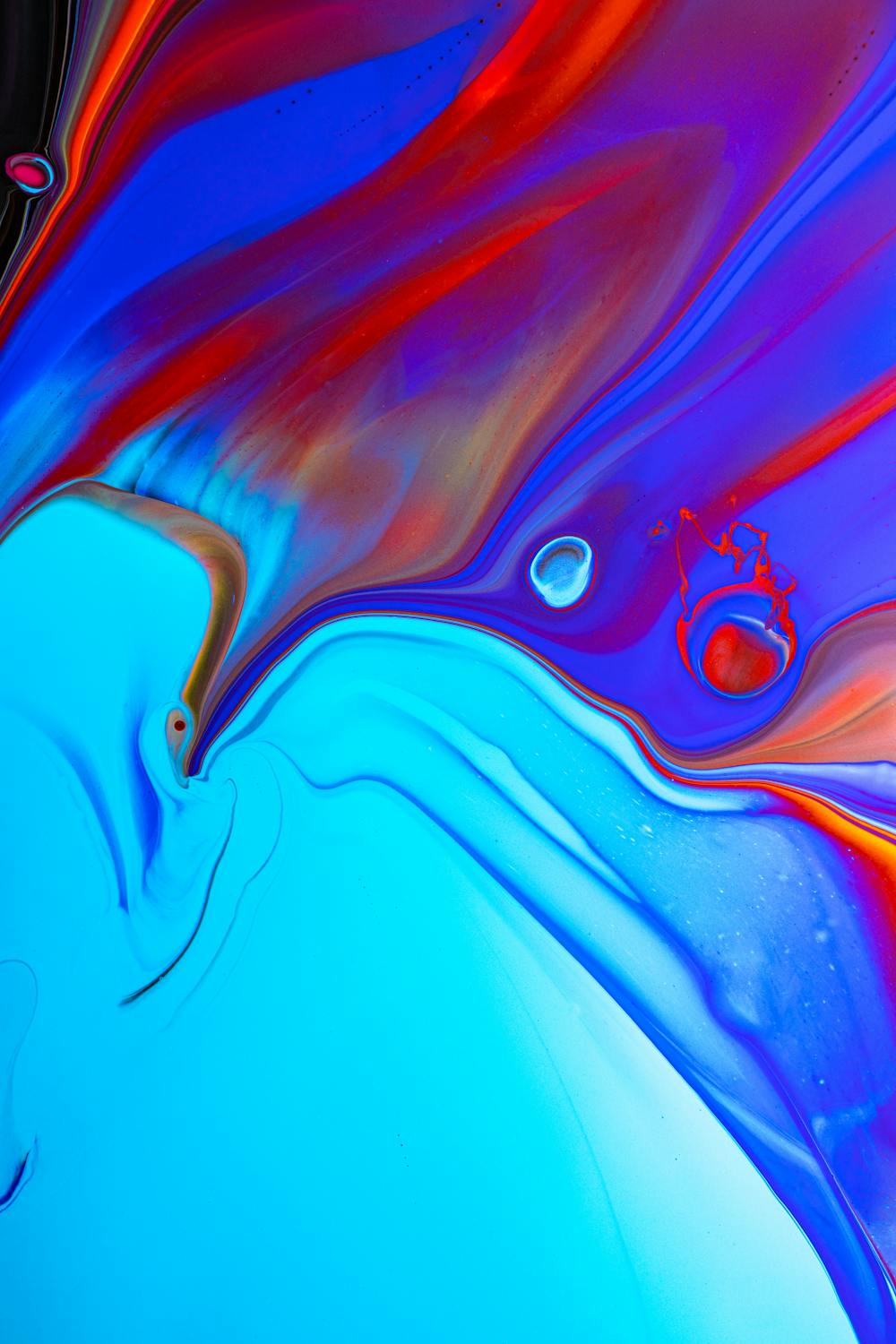