Transforming Health Insurance Fraud Detection With AI-Driven Anomaly Detection

Background
The health insurance sector in Saudi Arabia faces significant challenges due to pervasive fraudulent activities. These practices result in substantial financial losses for insurance providers and undermine trust within the healthcare system. The lack of effective fraud detection mechanisms enables such fraudulent behaviors, inflating costs for providers and consumers alike. Addressing these issues requires implementing advanced AI-driven solutions to detect and prevent fraudulent health insurance claims. This project aims to ensure financial stability, promote transparency, and uphold the integrity of the Saudi Arabian healthcare industry.
Objective
The primary goal of this project was to develop a proof of concept (PoC) using AI models to detect fraud patterns in the health insurance sector of Saudi Arabia. Key objectives included:
- Building robust AI models to identify fraudulent activities.
- Utilizing client-provided or open-source datasets for analysis.
- Designing custom loss functions to minimize cost implications.
- Evaluating model performance with relevant metrics such as F1 Score, Recall, and Precision.
Approach
To address the challenges of health insurance fraud, the project followed a structured approach:
- Data Collection and Cleaning:
- Leveraged either client-provided datasets or open-source datasets.
- Focused on preparing clean and reliable data for analysis.
- Data Analysis and Feature Engineering:
- Tailored analysis techniques to identify specific fraud patterns and anomalies relevant to Saudi Arabia’s insurance landscape.
- Developed relevant features to enhance model accuracy.
- Model Development and Training:
- Built and tested multiple AI models to detect anomalies in health insurance claims.
- Integrated advanced techniques, such as custom loss functions, to minimize the financial impact of misclassifications.
- Model Evaluation:
- Measured model effectiveness using industry-standard metrics like F1 Score, Precision, Recall, and Confusion Matrix.
Results and Impact
The project successfully developed a proof-of-concept AI system capable of detecting fraudulent health insurance claims. Key outcomes included:
- Enhanced detection accuracy, reducing false positives and negatives.
- Development of tailored fraud detection solutions for the Saudi insurance industry.
- Improved financial efficiency for insurance providers by curbing fraudulent activities.
- Promoted trust and transparency within the healthcare system, benefiting honest policyholders.
The results demonstrated that AI-driven fraud detection could significantly reduce financial losses and enhance operational integrity in the health insurance sector.
Future Implications
This project underscores the potential of AI in transforming fraud detection for health insurance. Future implementations could:
- Influence policy-making to adopt AI-driven fraud prevention methods.
- Expand the AI framework to other sectors facing similar challenges.
- Incorporate real-time fraud detection systems for proactive prevention.
- Stimulate further research into AI applications for healthcare and insurance, addressing global challenges.
By leveraging these findings, stakeholders can build a more transparent and resilient health insurance ecosystem, ensuring long-term benefits for providers and consumers alike.
This challenge is hosted with our friends at



Become an Omdena Collaborator
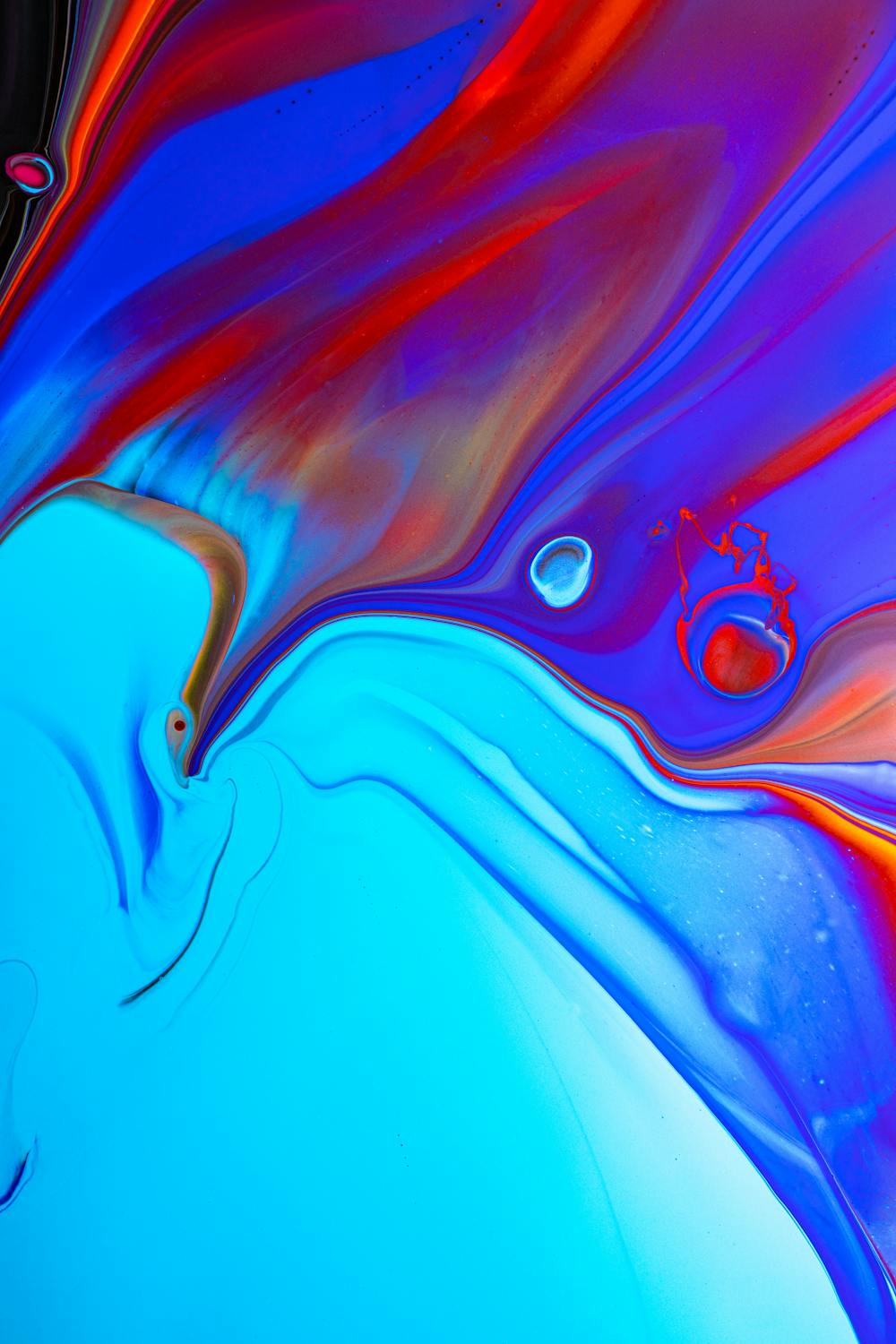