Predicting Painful Movements For Elbows Using Machine Learning

In this 8-weeks challenge, 50 AI change-makers collaborated to help assess, and normalize elbow pain monitored data and predict elbow painful behaviors to avoid.
The problem
Lateral elbow pain is one of the most common sources of medical consultation for nontraumatic elbow disorders. The most frequent diagnosis is lateral epicondylitis (LE), also known as tennis elbow. Strain in the forearm extensors of the fingers and the wrist is the principal factor that leads to developing this kind of injury. However, social-demographic, physical, psychological, diseases, and work-related factors are also associated with the underlying likelihood of developing this kind of musculoskeletal injury.
Remote assessment with MLI® Elbow from PRECURE helps to prevent strain in the forearm muscles and therefore reduces the risk of developing the injury. On the one hand, MLI® Elbow is an intelligent wearable that measures muscle strain by using EMG signals, which represent the contraction and relaxation of the muscles. On the other hand, the MLI® technology analyses these EMG signals using risk thresholds based on research and provides an MLI (Muscle Load Index) on a scale from 0 to 10.
Therefore, using the MLI, the user is informed immediately when the load is harmful and “nudged” to change behavior. Doing so can help to prevent wear and tear. Effective behavioral change empowers the user, reduces healthcare utilization, and, most importantly, reduces pain. Moreover, if this solution is applied to the labor sphere, it provides a healthier workplace and reduces costs and loss of productivity.
The project goals
1. Reliable normalized EMG
Surface EMG signal levels vary from person to person due to physiological differences, like skin, the muscular mass, fat… or slightly different electrodeposition. Lifting a load of 5kg can produce a signal of 2 mV for someone and a signal of 1,8 mV for another. Fortunately, for the same person, EMG and load are positively correlated.
The challenge is to normalize the EMG signals to be able to compare EMG activity in the same muscle on different days or in different individuals. Currently, we are using Maximum (peak) activation levels during maximum contractions for EMG normalization. However, this peak value might not be obtained using the best practices like avoiding outliers and noise.
2. Predict painful behavior
We have observed how, by providing visual feedback with the MLI, users have experienced a reduction in pain because of changing behavior. The challenge is to investigate if there exist strain patterns (EMG) prior to pain and therefore predict when this pain can arise again. Pain in the elbow is subjectively evaluated and reported by the users in a Visual Analog Scale (VAS) that ranges from 0 (no pain) to 10 (worst imaginable pain). In the case of “tennis elbow,” a prediction for pain or no pain for an injured user is enough. However, the VAS might be helpful to know if this pain increases or decreases. Individual attributes can also be used in the model.
First Omdena Project?
Join the Omdena community to make a real-world impact and develop your career
Build a global network and get mentoring support
Earn money through paid gigs and access many more opportunities
Your benefits
Address a significant real-world problem with your skills
Get hired at top companies by building your Omdena project portfolio (via certificates, references, etc.)
Access paid projects, speaking gigs, and writing opportunities
Requirements
Good English
A very good grasp in computer science and/or mathematics
Student, (aspiring) data scientist, (senior) ML engineer, data engineer, or domain expert (no need for AI expertise)
Programming experience with Python
Understanding of Data Engineering, Data Analytics, and Machine Learning.
This challenge is hosted with our friends at
Application Form



Become an Omdena Collaborator
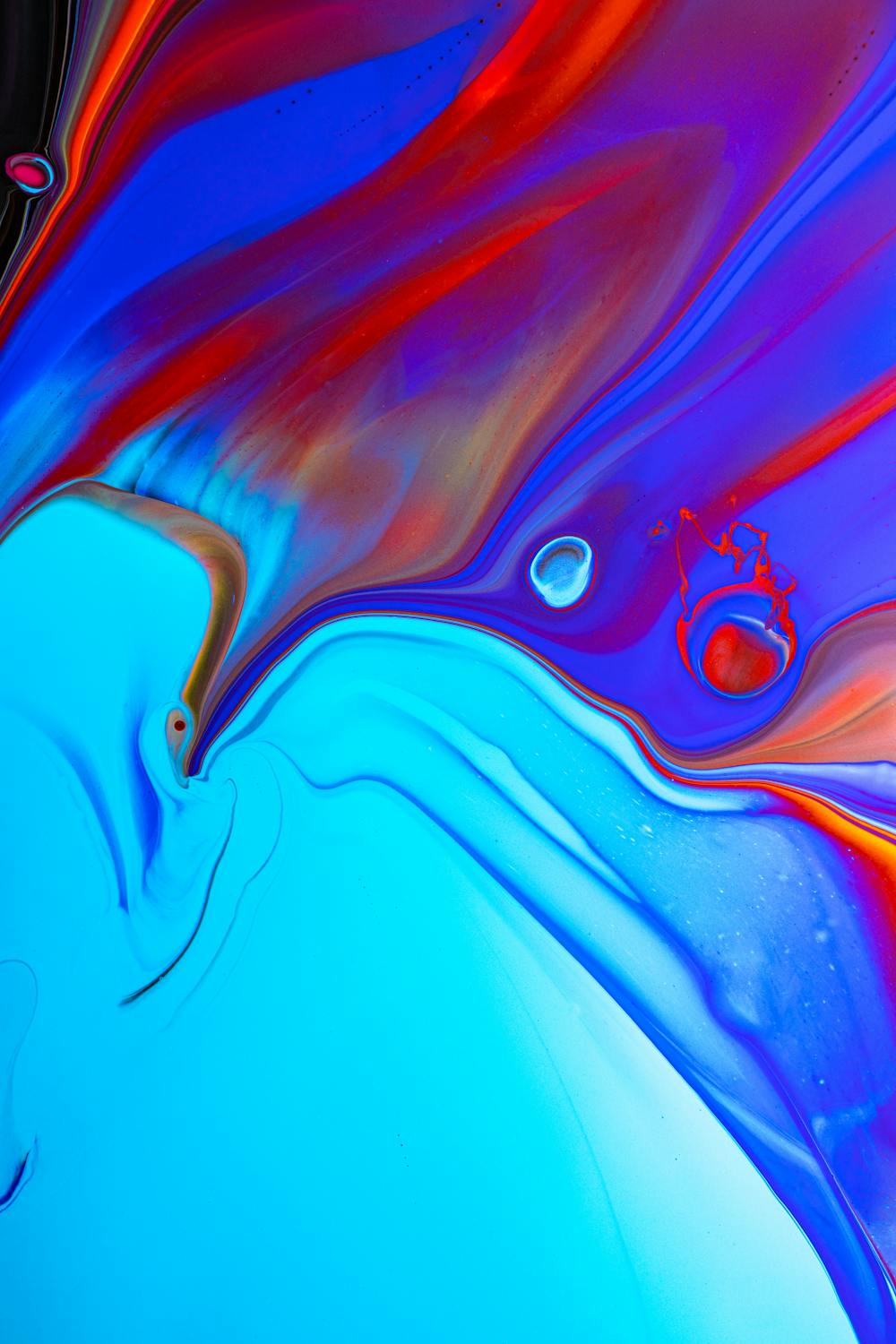