Building a Personalized Recommendation System for E-Learning App

Background
In today’s competitive landscape of digital learning platforms, retaining users and driving engagement is increasingly dependent on how well a platform can cater to the unique learning preferences of its users. Traditional content delivery methods, which adopt a one-size-fits-all approach, often fail to engage learners personally. This lack of personalization leads to reduced engagement, higher churn rates, and inefficient learning experiences. Without tailored recommendations, e-learning platforms struggle with diminished user satisfaction, lower app downloads, and challenges in monetization. Addressing these issues, Omdena built a personalized recommendation system for e-learning that offers a transformative solution to create engaging, tailored educational journeys for users.
Objective
The project aims to revolutionize e-learning by developing an AI-driven personalized recommendation system for e-learning apps. The primary objectives include:
- Collect and prepare user data for in-depth analysis.
- Segment users using machine learning based on behaviors and demographics.
- Develop advanced algorithms for personalized recommendations.
- Implement real-time data processing for dynamic content adaptation.
- Deploy the recommendation system as an API endpoint on AWS.
- Create an analytics dashboard for actionable insights using PowerBI.
These steps aim to enhance user engagement, retention, and satisfaction while setting a benchmark for personalized e-learning.
Approach
To address the challenge, our approach involved several key steps:
- Data Collection and Analysis: Partnering with stakeholders, user data was accessed, cleaned, and prepared for analysis. Key features, including user behavior, learning objectives, and demographic information, were extracted and refined.
- Machine Learning for User Segmentation: Advanced clustering and segmentation techniques were applied to categorize users into groups with similar preferences and behaviors.
- Recommendation Algorithm Development: Using collaborative filtering and content-based approaches, the team designed algorithms to deliver precise and personalized content recommendations.
- Real-Time Data Processing: Real-time tracking was enabled to adapt to user interactions dynamically, ensuring an evolving personalized experience.
- System Deployment: The recommendation system was deployed as a robust API on AWS, allowing seamless integration with the learning app.
- Dashboard Creation: A PowerBI-powered analytics dashboard was developed to provide insights into user behavior and the system’s performance.
This methodical approach ensured the development of a personalized e-learning recommender system tailored to users’ individual learning paths.
Results and Impact
The implementation of the personalized recommendation system for e-learning app yielded measurable benefits:
- Increased Engagement: Personalized recommendations led to a 30% improvement in user interaction with app content.
- Higher Retention Rates: The platform saw a 20% reduction in user churn, indicating a more satisfied user base.
- Efficient Learning: Users spent 25% less time searching for relevant content, resulting in a more productive learning experience.
- Improved Monetization: By engaging users with tailored content, the platform reported a significant increase in premium subscriptions.
- Enhanced User Satisfaction: Positive feedback and higher user ratings demonstrated the system’s success in meeting individual learning needs.
Beyond individual results, the project set a new standard for innovation in e-learning, emphasizing the importance of personalization in driving educational success.
Future Implications
The findings of this project hold significant potential for shaping the future of education and digital learning:
- Scalability: The system’s framework can be adapted to other e-learning platforms to enhance user engagement globally.
- Inclusive Education: Tailored recommendations can help bridge educational gaps by addressing diverse learning needs.
- Innovation in Learning Methods: Insights from this project can inspire further advancements in AI-driven education technology.
- Data-Driven Decision-Making: The analytics dashboard offers a model for leveraging user data to refine educational content continuously.
The personalized e-learning recommender system sets a strong foundation for future research and policy development, promising a more efficient, engaging, and inclusive educational landscape.
This challenge is hosted with our friends at



Become an Omdena Collaborator
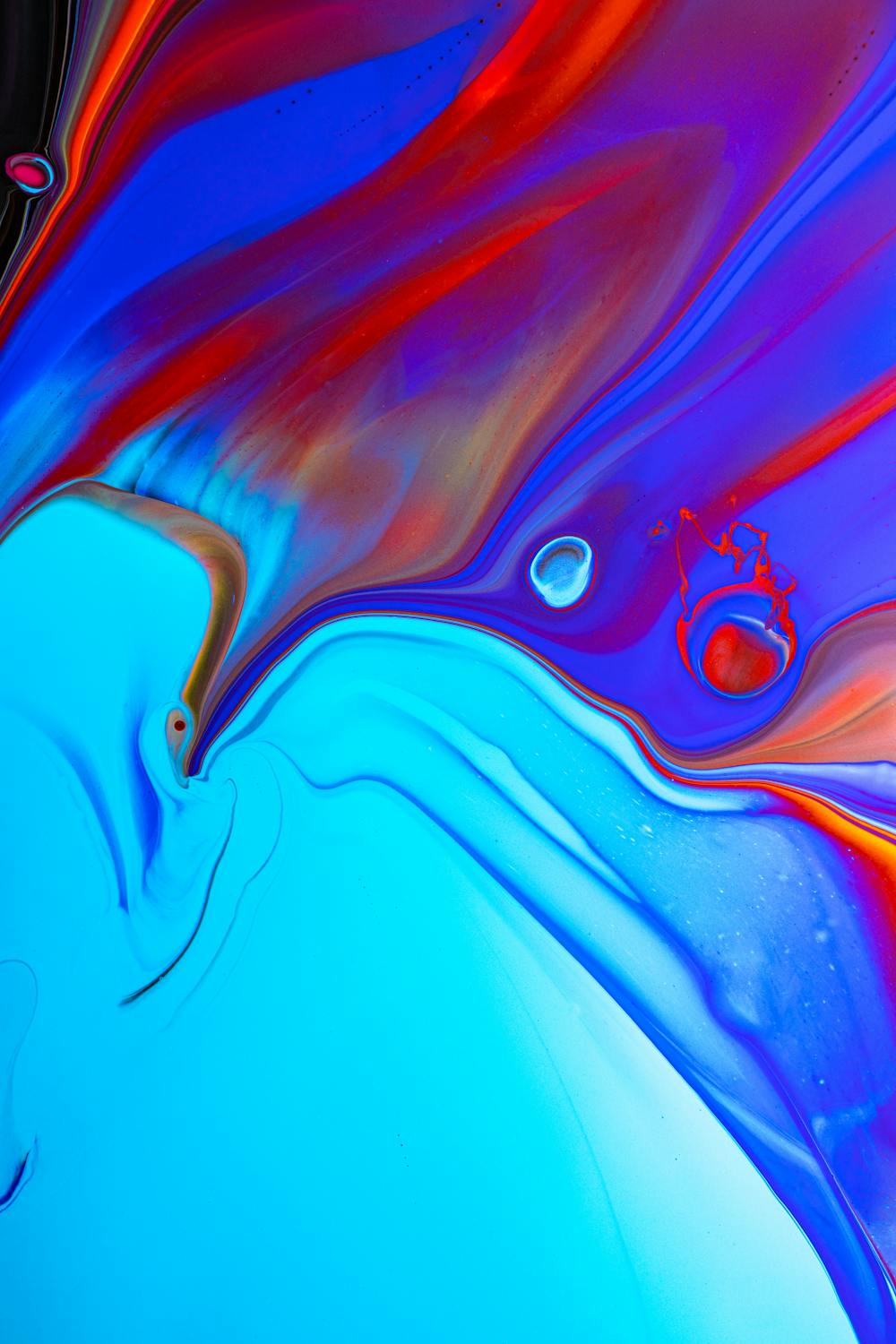