Detecting Pathology Through AI in Ultrasound Images

Background
Healthcare systems in under-resourced regions, particularly in Africa, face immense challenges, with limited access to medical expertise and diagnostic tools. Pneumonia is the leading cause of death in children under five, while breast cancer is the most diagnosed cancer among women, with growing incidence rates in African countries. Ultrasound imaging, being portable, cost-effective, and non-invasive, offers immense potential for diagnosing life-threatening conditions in such contexts. However, integrating Artificial Intelligence (AI) into ultrasound imaging can elevate its effectiveness, delivering radiology-level precision for detecting and localizing pathologies, even in resource-constrained environments.
Objective
The project aims to develop an AI-powered Ultrasound solution capable of:
- Detecting multiple pathologies across 2D images and video streams.
- Identifying the location of these pathologies with high accuracy.
- Making advanced diagnostic tools accessible to underserved regions, thereby democratizing healthcare access.
Approach
Envisionit Deep AI, in collaboration with Omdena and 50 technology changemakers, implemented a structured, multi-phase approach:
- Image Preprocessing and Normalization: Diverse ultrasound datasets in varying formats and resolutions were normalized to ensure consistency. Image normalization routines were optimized to align with clinical production environments.
- Model Training: Custom AI algorithms were designed to detect and localize over 10 predefined pathologies, with the capability to scale further. Advanced object detection techniques were employed to pinpoint pathology locations within images or video frames.
- Model Validation and Specialist Review: Performance metrics were rigorously analyzed. Radiologists conducted independent validations using external datasets to ensure an accuracy rate of at least 95%. Only models with combined automated and expert-validated accuracy exceeding 98% were deemed ready for deployment.
- Field Deployment with Feedback Integration: AI models were deployed with built-in feedback mechanisms for real-time adjustments. Radiologists and field users could refine AI predictions by adding or modifying detected pathologies, ensuring continual model improvement.
- Concordance/Discordance Review: A radiologist validated all feedback to maintain the integrity of training datasets. Automation tools facilitated quorum testing, prioritizing feedback requiring human review, and preventing data validation bottlenecks.
Results and Impact
- The AI solution achieved over 98% accuracy in pathology detection and localization.
- Field pilots demonstrated enhanced diagnostic capabilities, enabling faster, more accurate medical interventions.
- The portable, AI-enabled Ultrasound tool empowered clinicians in resource-limited settings, significantly improving disease detection rates for conditions like pneumonia and breast cancer.
This transformative AI Ultrasound solution not only addresses the immediate diagnostic needs of under-resourced healthcare systems but also bridges the gap in healthcare equity.
Future Implications
This pioneering work lays the groundwork for:
- Broader integration of AI in Ultrasound imaging technology, facilitating diagnosis of a wider range of diseases.
- Scaling the solution globally to address healthcare disparities, particularly in low-resource regions.
- Informing future policies and healthcare innovations that leverage AI for disease detection and telemedicine practices.
By combining cutting-edge ultrasound imaging technology with AI, Envisionit Deep AI and Omdena are shaping a future where accurate, accessible, and affordable healthcare is a reality for all.
This challenge is hosted with our friends at



Become an Omdena Collaborator
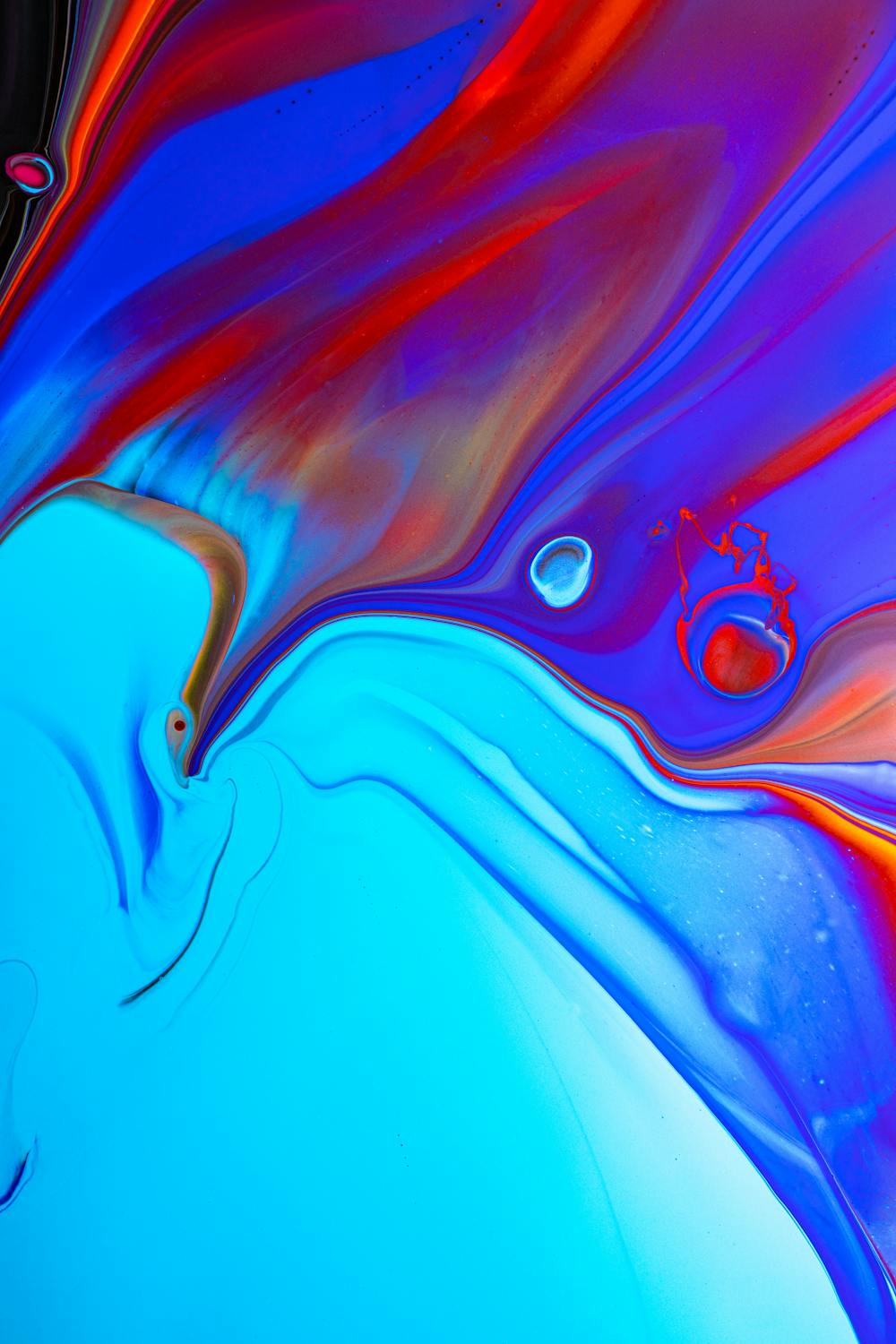