Sleep Pose Estimation Using AI to Improve Sleep Apnea Condition

Background
Globally, over 1 billion people suffer from sleep apnea, a condition marked by repeated interruptions in breathing during sleep. The most common cause, Obstructive Sleep Apnea (OSA), results from the relaxation of throat muscles blocking the airways. Research indicates that sleep position significantly influences the severity and frequency of OSA episodes. Addressing this challenge, Sleepiz AG introduced a contactless medical device using continuous wave radar technology to monitor sleep movements with sub-millimeter precision.
Objective
The primary goal of this project was to develop AI algorithms capable of accurately estimating sleep poses to assist in diagnosing and treating sleep apnea, particularly for patients with positional sleep apnea.
Approach
This high-impact 8-week challenge involved 50 AI engineers working collaboratively to solve the problem. The team utilized:
- Data Sources: I/Q radar-based time series data and complex Electromyography (EMG) data.
- Techniques: Machine learning algorithms to analyze periodic limb movements and detect sleep poses.
- Tools: Advanced AI and machine learning frameworks were used to process and interpret radar and EMG data.
Results and Impact
The project successfully developed multiple machine learning algorithms to detect periodic limb movements and accurately estimate sleep poses. These innovations contribute to:
- Enhanced diagnostic capabilities for sleep apnea patients.
- Improved treatment strategies tailored to patients with positional sleep apnea.
- A scalable solution for integrating contactless monitoring devices in sleep healthcare, potentially benefiting over 1 billion people globally.
Future Implications
The findings from this project pave the way for:
- Further development of AI-based sleep monitoring systems.
- Enhanced treatment protocols for sleep apnea based on precise sleep pose analysis.
- Broader adoption of contactless medical devices in healthcare, improving accessibility and patient comfort.
By advancing the understanding of sleep pose estimation, this work could influence both clinical practices and future research in sleep health technologies.
This challenge has been hosted with our friends at



Become an Omdena Collaborator
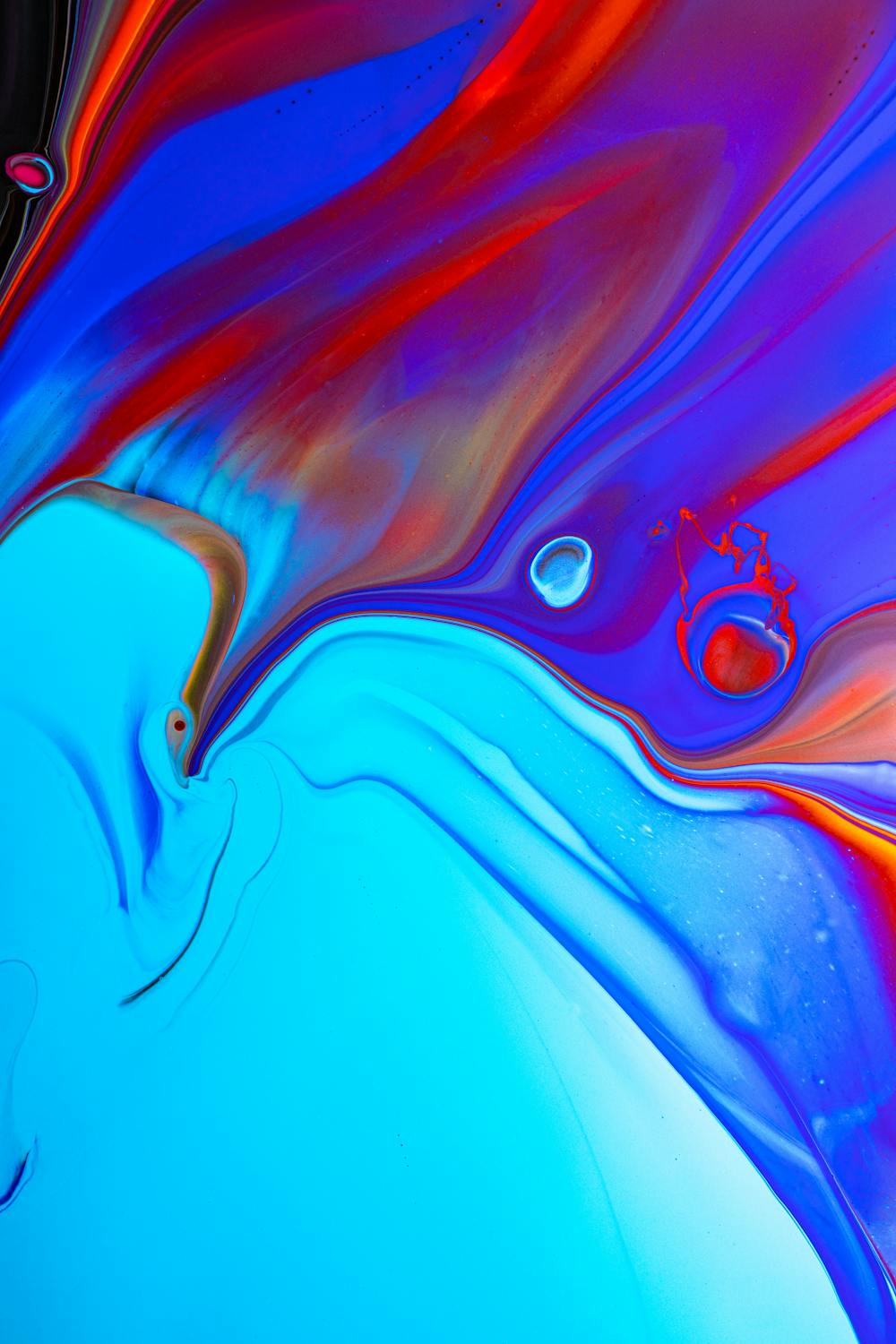