Detecting Malnutrition in Children Using AI and Computer Vision

Malnutrition in children
Background
Malnutrition remains a leading global health challenge, affecting over 200 million children under the age of five. It is a significant contributor to child mortality, with approximately 3 million preventable deaths annually. Traditional anthropometric methods for malnutrition diagnosis are often complex, time-consuming, and prone to error, making them unsuitable for large-scale application. The Child Growth Monitor (CGM) initiative sought to address these challenges by developing an innovative AI-based system to provide precise, scalable, and cost-effective malnutrition detection
Objective
The main goal of the malnutrition detection project was to enhance the accuracy of CGM’s neural network predictions to ensure that 90% of children’s height measurements have an error margin of less than 1cm. This improvement aims to provide reliable and quick assessments to prevent malnutrition-related fatalities.
Approach
Omdena’s collaborative platform engaged 61 AI changemakers to develop and refine CGM’s AI system. Key aspects of the project included:
- Data Integration: Leveraging datasets (PCD, RGB images, and CSVs) based on WHO Child Growth Standards, including both cleaned and real-world data from diverse environments.
- Model Development: Training neural networks to predict critical metrics like height, weight, and mid-upper arm circumference (MUAC) from 3D scans.
- Artifact Selection: Filtering scan data (RGB images and depth maps) to identify the most reliable inputs for accurate results.
- Reliability Measures: Introducing automated checks for errors, such as incomplete scans or poor lighting, and implementing confidence intervals for measurement predictions.
- Validation and Testing: Ensuring the AI solution performs effectively across varied conditions without manual intervention while safeguarding against measurement errors
Results and Impact
The project significantly improved CGM’s performance:
- Increased measurement accuracy to ~60% for real-world scans after manual cleaning.
- Developed robust algorithms capable of detecting malnutrition indicators under diverse conditions.
- Automated error detection mechanisms to prevent inaccurate diagnoses.
This AI-powered tool enhances early malnutrition detection, enabling timely interventions and potentially saving millions of lives globally.
Future Implications
The malnutrition detection project lays the groundwork for scalable AI applications in global health. Potential future advancements include:
- Expanding the model to incorporate additional health indicators, such as stunted growth or obesity.
- Scaling deployment to regions with high malnutrition rates through partnerships with NGOs and governments.
- Refining AI models with additional datasets for even greater accuracy.
The initiative serves as a model for using AI to address critical social challenges.
For more detailed insights and resources, refer to the CGM´s GitHub repository.
Learn more about Child Growth Monitor
This challenge is hosted with our friends at



Become an Omdena Collaborator
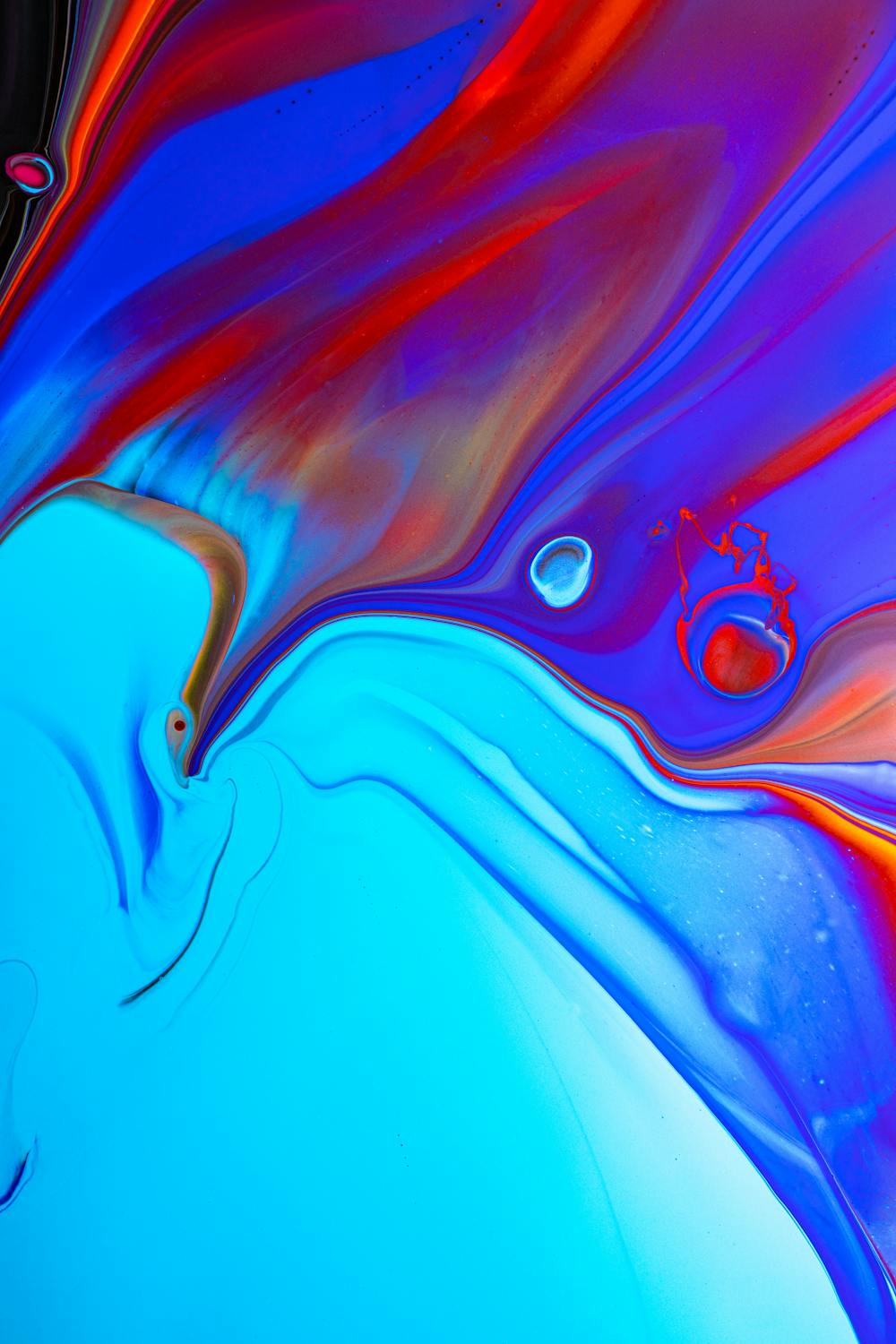