Understanding Land Ownership in Kenya Through NLP to Help Restore Lands

Background
Monitoring environmental changes globally is challenging, requiring the analysis of biophysical changes alongside social changes like policy shifts and grassroots movements. Understanding the hidden actors and interests behind land management is crucial for tackling key barriers to forest and landscape restoration, combating corruption, and fostering sustainability. Limited insight into these networks hampers the global development community’s ability to implement effective action plans.
To address these challenges, Code for Africa, Stanford University, and the World Resources Institute partnered to analyze publicly available documents in Kenya. Using Natural Language Processing (NLP), the team sought to map connections within open-source land ownership documents, aiming to bolster transparency and equity in land restoration efforts.
Objective
The project aimed to:
- Create a knowledge graph of land ownership in Kenya.
- Generate a “Watch List” of organizations to identify potential corruption in land ownership.
- Map networks from open-source land documents to polygons, enabling geo-location of land and associated actors.
Approach
The project built on a pilot created by Stanford University’s Data Science for Social Good cohort, which utilized Kenya’s public land ownership records, such as government gazettes. The team extended this work by:
- Using data sources like the connectedAFRICA project, sourceAFRICA repository, and gazeti.AFRICA digitization campaign.
- Analyzing court records, government procurements, and other public documents with NLP techniques.
- Mapping connections and networks within open-source land documents.
- Cross-referencing findings with “Watchlist” data to identify politically exposed persons and organizations potentially involved in corruption.
Results and Impact
The project delivered a comprehensive knowledge graph of land ownership in Kenya, uncovering intricate networks among individuals and organizations. The following outcomes were achieved:
- Identification of corruption patterns through a detailed “Watch List.”
- Enhanced capacity for journalists, researchers, and investigators to promote good governance.
- Insights into land ownership networks, supporting Kenya’s efforts to restore degraded lands equitably.
These findings contribute to sustainable land management and provide a framework for combating corruption, ultimately fostering transparency and accountability in natural resource management.
Future Implications
This project lays the groundwork for advancing policies and research in land management across Africa. The methodology developed here can scale to other regions, enabling broader adoption of knowledge graphs for natural resource governance. By identifying corruption and fostering transparency, this initiative can significantly influence future restoration efforts and global sustainability strategies.
This challenge has been hosted with our friends at



Become an Omdena Collaborator
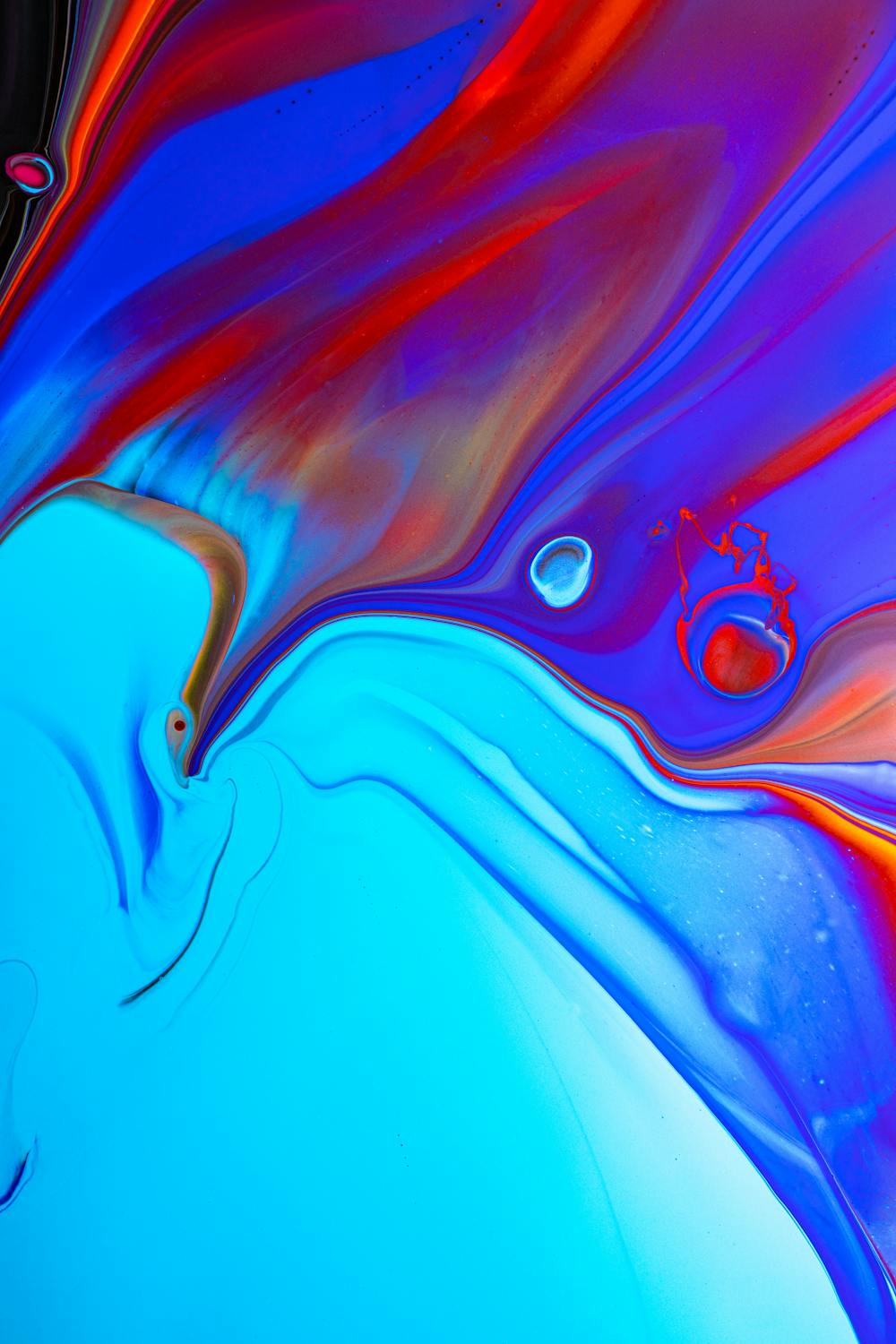