Identifying Floor Plan Symbols Using Computer Vision

Background
Creating city permit-compliant energy model reports traditionally demands extensive manual labor—up to three months for a team of 4–5 experts. This prolonged process not only incurs significant costs but also deters real estate developers from prioritizing emission reduction. Smaller developers, in particular, face barriers due to the high financial and time requirements. Addressing these challenges, the Omdena team collaborated with Sysconverge Inc., a Canada-based data analytics firm, to leverage artificial intelligence for automating the identification of building elements/symbols in 2D floor plans. This innovation enhances efficiency and contributes to sustainable architecture practices.
Objective
The project aimed to:
- Develop a computer vision model capable of identifying diverse building elements—such as walls and railings—in 2D floor plans.
- Facilitate the creation of energy model reports for real estate developers.
- Reduce the cost and time required for energy modeling, enabling a focus on emission reductions and making the process accessible to smaller developers.
Approach
The Omdena team employed the following methods to tackle this problem:
- Data Collection: Leveraged Sysconverge’s datasets and additional sources to annotate over 150 objects across various 2D floor plan images.
- Object Detection: Implemented computer vision techniques to accurately identify floor plan symbols and classify elements such as walls, doors, and railings.
- Semantic Segmentation: Utilized advanced AI models to perform detailed detection and dimension estimation, creating a structured digital representation of floor plans.
- Collaboration: Engaged a global team of AI experts to ensure comprehensive problem-solving and innovation.
Results and Impact
- Enhanced Accuracy: Successfully developed a model capable of identifying and dimensioning over 150 types of building elements with precision.
- Time and Cost Savings: Reduced the effort required for manual energy model reporting, potentially cutting the process duration by months.
- Environmental Benefits: Enabled developers to focus on emission reduction by simplifying the compliance process.
- Increased Accessibility: Lowered barriers for small-scale real estate developers, fostering inclusivity in sustainable building design.

Project screenshot: Floor plan labeling.
Future Implications
The success of this initiative highlights several opportunities for the future:
- Policy and Compliance: Streamlined processes for creating energy reports can become an industry standard, encouraging widespread adoption of sustainable practices.
- Architectural Innovation: AI-powered tools can inspire new design methodologies, enhancing creativity and efficiency.
- Sustainability: Integrating digitized floor plans into energy modeling can further reduce carbon footprints, supporting global climate goals.
- Research and Development: Expanding the dataset and refining AI models can lead to broader applications, such as urban planning and infrastructure monitoring.
This project demonstrates how AI-driven solutions can revolutionize the architecture and construction industries, driving sustainability and innovation while reducing operational costs.
This challenge is hosted with our friends at



Become an Omdena Collaborator
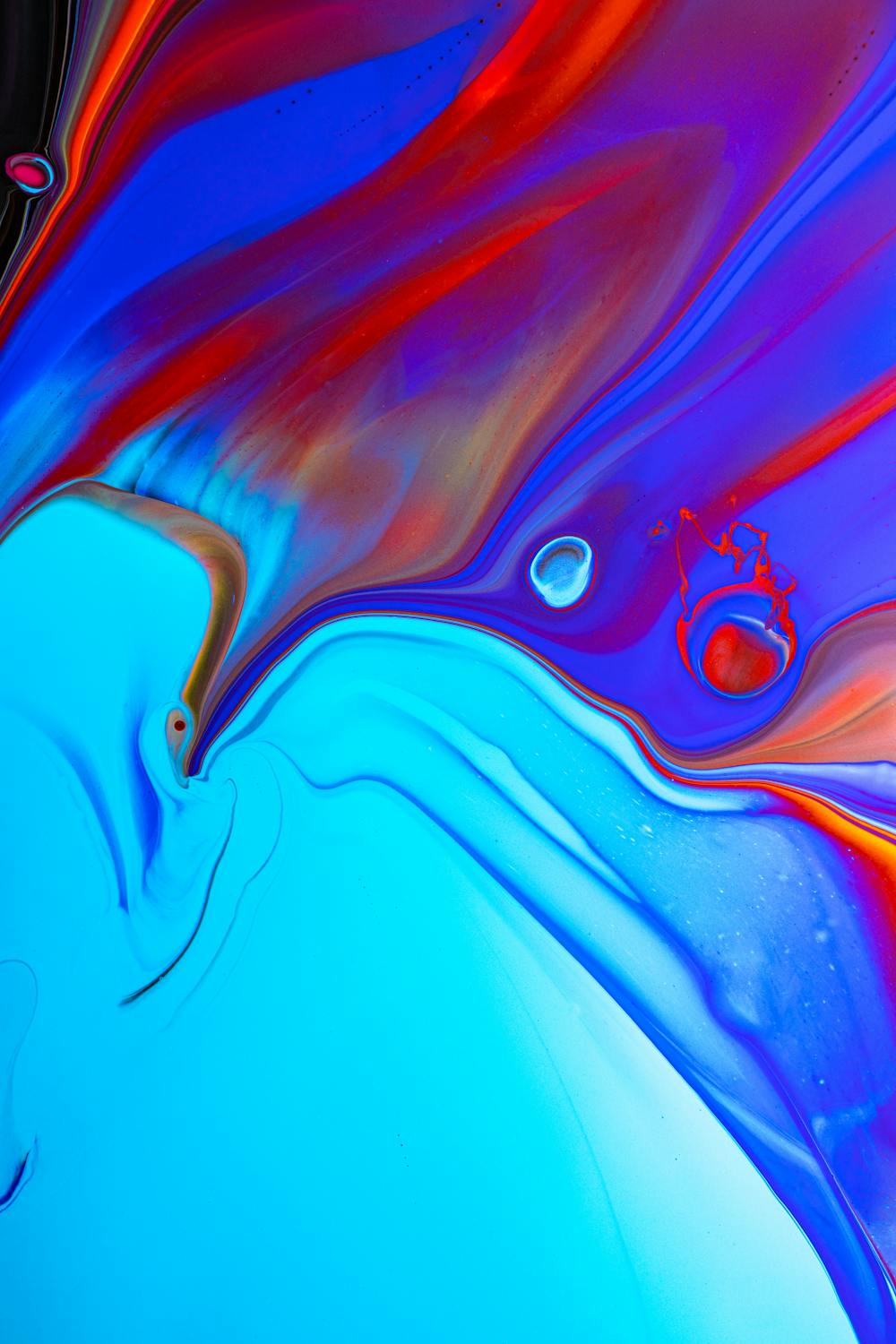