Optimizing Smart Electric Vehicle (EVs) Charging Network Management Using AI and Machine Learning

Background
The rapid adoption of electric vehicles (EVs) presents significant challenges to existing energy networks, primarily due to unexpected peak loads and voltage issues. Understanding EV charging behaviors is crucial for designing effective charging incentives and energy management strategies. Leveraging smart meter data, AI and machine learning offer a non-intrusive solution to track charging patterns, enabling Distributed Network Service Providers (DNSPs) to enhance network planning and operations.
Objective
The primary goal of this project was to analyze EV charging data using machine learning to optimize Distributed Network Service planning. This involved identifying consumption patterns and peak charging times, helping system operators manage and improve the efficiency of EV charging operations.
Approach
- Data Preparation: Significant effort was dedicated to cleaning and organizing smart meter data for analysis.
- Exploratory Data Analysis (EDA): The team conducted EDA to understand consumption patterns among different user types, such as EV users and peak consumers.
- AI Modeling: A clustering algorithm was used to categorize consumption data into three clusters (low, medium, high) to identify potential EV charging events.
- Tool Development: Insights were compiled into an Excel tool and dashboard for easy access by system operators.

Figure 1: Average hourly consumption for one day for EV and non-EV users
Results and Impact
- Pattern Identification: The project successfully identified when and how much EVs are typically charged, along with peak consumption periods.
- Network Management: Insights from the project enable DNSPs to manage their networks more effectively, optimizing the efficiency of EV charging operations.
- Decision Support: The Excel tool and dashboard provide actionable insights for system operators, aiding in strategic planning and operations.
Future Implications
The findings from this project can guide future policies on energy management and EV charging incentives. As more data becomes available, machine learning models can be refined further to improve accuracy and predictive capabilities. This approach can also be extended to other regions or adapted for different types of energy networks, contributing to more sustainable energy management practices globally.
This challenge is hosted with our friends at



Become an Omdena Collaborator
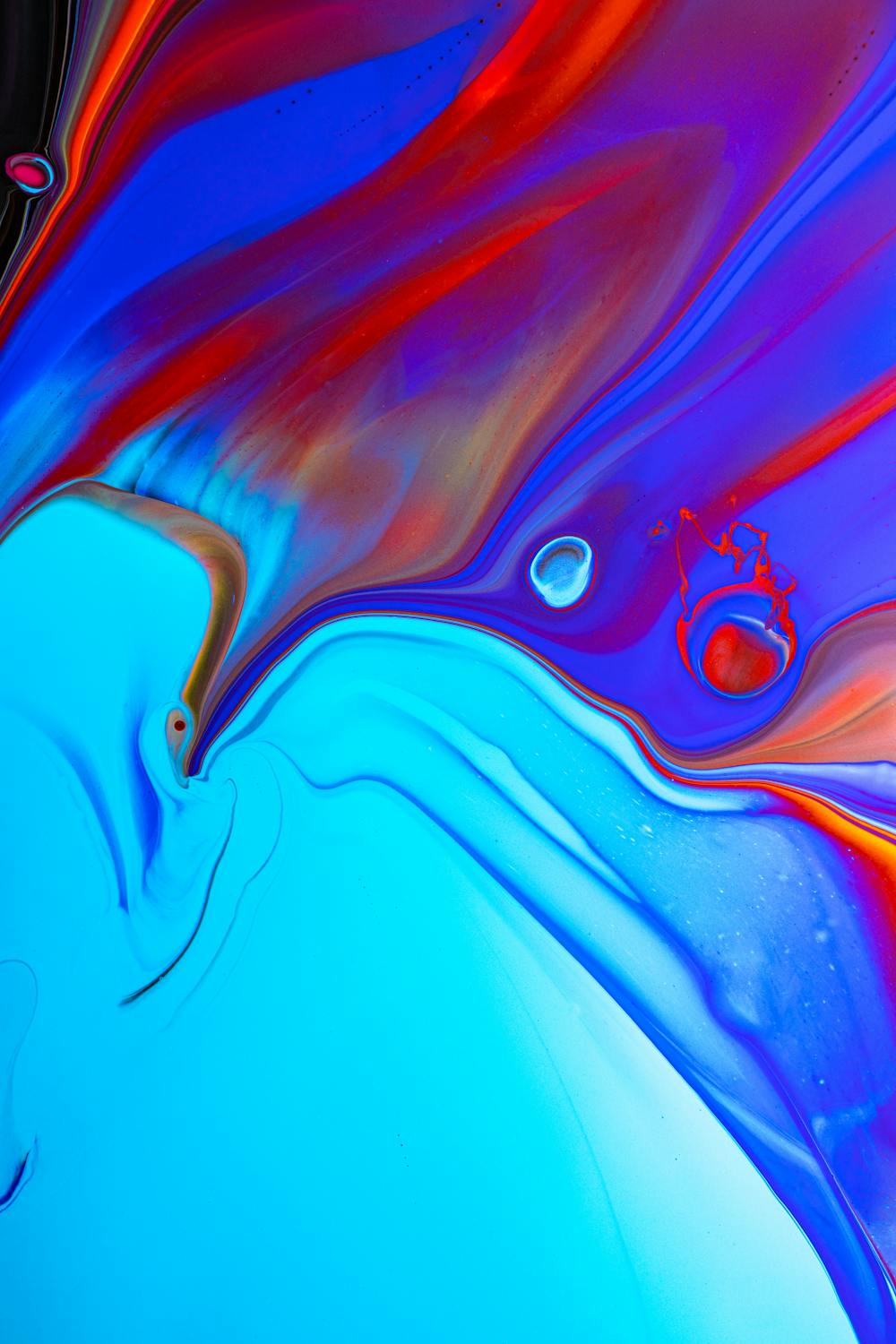