Increasing Drug Safety By Detecting Anomalies in Clinical Data Using Machine Learning

Background
The exponential growth of real-world clinical data poses significant challenges in reliability and synchronization. Data is generated continuously from diverse sources at varying times, leading to unsynchronized and sometimes inaccurate records. For a $1.5 trillion/year life sciences industry, where data mismanagement can affect outcomes, these challenges raise critical questions: Can this data be trusted for decision-making? How can risks be quantified, especially in scenarios like vaccine trials where errors could impact millions?
Objective
The project aimed to develop an anomaly detection API for clinical data. This API is designed to enhance the safety and efficacy of medical devices and drugs by identifying inconsistencies in clinical trial and connected device data. The solution empowers life sciences companies to manage clinical data more effectively and ensures independent validation of decisions made using healthcare data.
Approach
The Omdena team collaborated with Flaskdata, an Israeli tech startup, to tackle the challenges of anomaly detection in clinical data. The team focused on two main use cases:
- Use Case 1: Clinical Trial Data
- Tackled the complexity of high-dimensional, sparse, and non-stationary clinical trial data.
- Used an ensemble approach combining:
- Detection of outliers in multidimensional data points.
- Time-series drifts and spikes.
- Therapeutic-user-specified rules (e.g., flagging suicidal tendencies in psychiatric studies).
- Addressed challenges such as lack of training sets, small datasets, and bias issues.
- Use Case 2: Connected Devices
- Focused on time-series data from wearable and medical devices.
- Evaluated device data for reliability, patient pre-screening, and adverse event monitoring.
- Utilized anomaly detection to identify patterns such as rising or dropping blood pressure indicative of serious adverse events.
- API Development
- Built a RESTful API capable of processing structured clinical data.
- Designed the algorithm to be model-free, scalable, and compatible with high-dimensional datasets.
Results and Impact
The project delivered a RESTful API for automated anomaly detection in clinical data, yielding several measurable benefits:
- Enhanced Safety: Improved identification of adverse events and patient safety issues.
- Data Reliability: Enabled better validation of clinical trial data.
- Efficiency: Facilitated quicker and more accurate clinical trials by automating anomaly detection.
- Accessibility: Democratized access to clinical data anomaly detection, making it available to smaller organizations and startups.
This solution contributes to safer medical devices and drugs, ultimately enhancing patient outcomes and public trust in healthcare.
Future Implications
The findings from this project have far-reaching implications:
- Healthcare Policies: Can inform policies to mandate independent anomaly detection in clinical data.
- Future Research: Provides a foundation for advanced anomaly detection techniques in various healthcare applications.
- Medical Devices: Enhances the integration of wearable and connected devices in clinical trials, improving patient monitoring and safety.
- Global Impact: Supports the growing demand for reliable clinical data in a digitized, data-driven healthcare ecosystem.
This challenge has been hosted with our friends at



Become an Omdena Collaborator
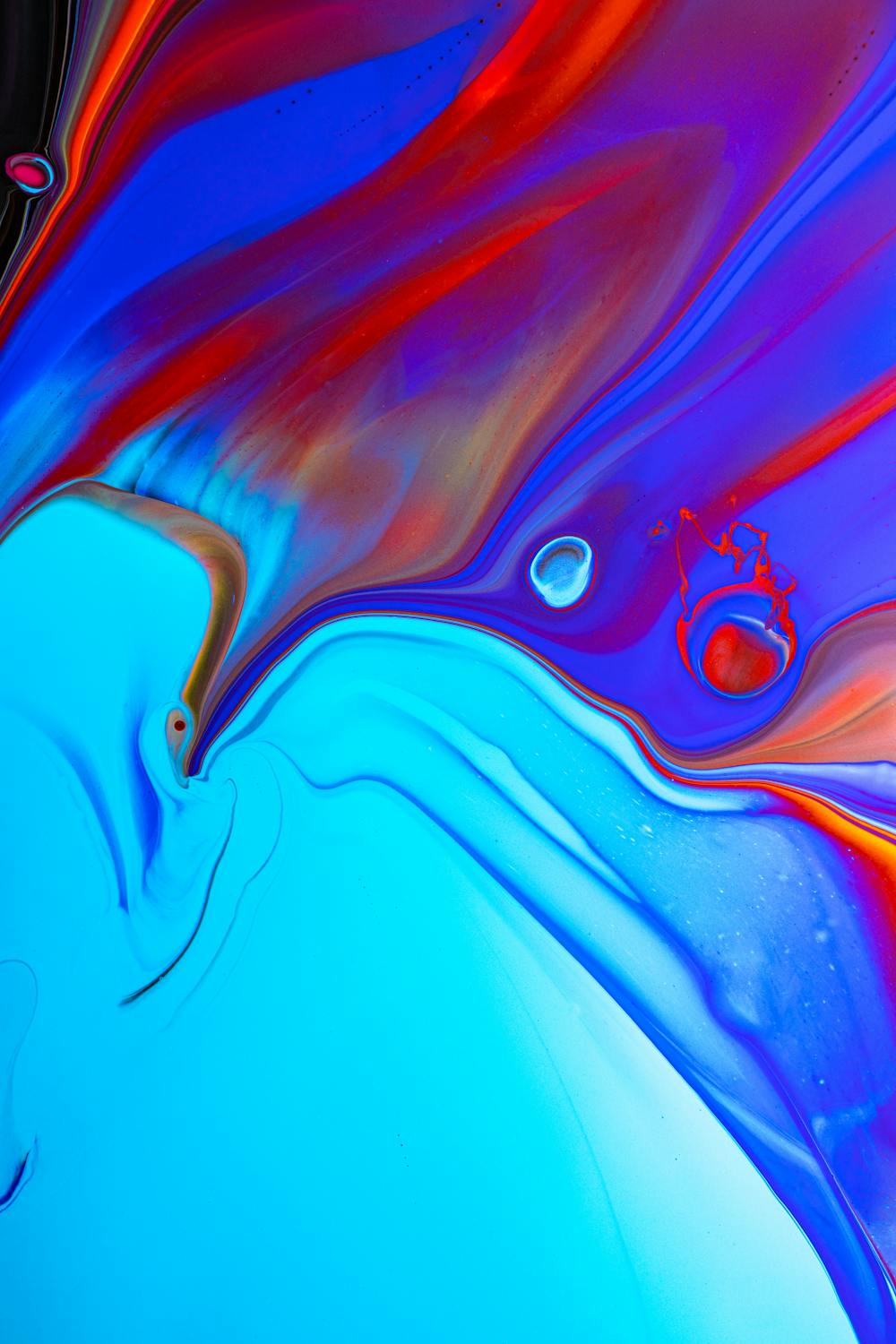