Identifying Economic Incentives for Forest and Landscape Restoration

Background
We are entering the United Nations Decade for Ecosystem Restoration (2021–2030), a global initiative to restore ecosystems and address climate change, biodiversity loss, and resource security. To prepare for this effort, understanding enabling policies and their incentives is crucial. However, analyzing thousands of policy documents across multiple sectors is a significant challenge. Using Natural Language Processing (NLP), this project sought to mine policy documents for actionable insights, promoting knowledge sharing and identifying economic and financial incentives, disincentives, and misaligned policies.
Objective
The project’s primary goals were:
- Identify policies related to forest and landscape restoration.
- Detect economic and financial incentives embedded in policy documents.
- Create a heatmap visualizing the relevance of policies across countries in Latin America.
Approach
The team utilized a comprehensive NLP pipeline to analyze policy documents from five Latin American countries: Mexico, Peru, Chile, Guatemala, and El Salvador. The process included:
- Data Collection: Scraping official policy databases and conducting Google scraping to gather thousands of relevant documents from 2016–2020.
- Data Filtering: Narrowing down documents based on relevance to forest and landscape restoration.
- Topic Modeling: Applying the Latent Dirichlet Allocation (LDA) algorithm to classify text fragments into policy topics.
- Visualization: Creating a heatmap to illustrate the distribution of policy topics by country, enabling cross-country comparisons.
Example visualization: Heatmap displaying the frequency of policy topics by country
Results and Impact
The project resulted in a robust database of policy documents and a heatmap visualization showcasing policy relevance to forest and landscape restoration across Mexico, Peru, and Chile. Key insights included:
- Identification of territorial-related policies prevalent in Mexico but less adopted in Chile and Peru.
- Enhanced understanding of policy distribution, helping policymakers make informed decisions.
- Evidence-based tools for stakeholders to advocate for policy changes to support ecosystem restoration.
These findings offer a data-driven approach to addressing environmental challenges, contributing to Sustainable Development Goals (SDGs).
Future Implications
The project’s methodologies and findings can shape future policies by providing:
- A standardized NLP approach for policy analysis.
- Data to advocate for filling gaps in economic incentives for restoration.
- A model for replicating similar studies in other regions.
By leveraging these insights, policymakers and stakeholders can implement effective, evidence-based strategies to achieve ecosystem restoration goals globally.
This challenge is hosted with our friends at



Become an Omdena Collaborator
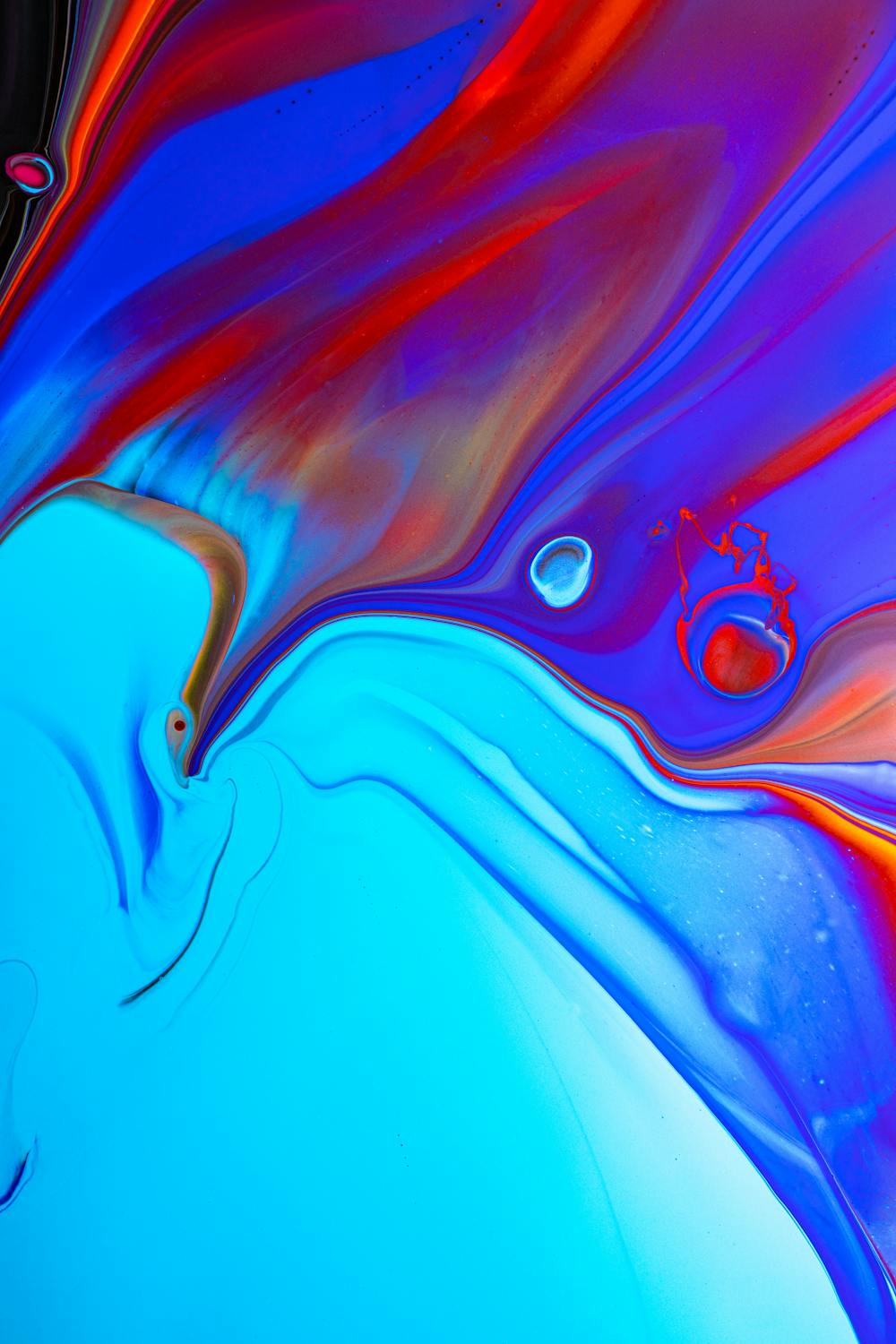