Improving Extreme Weather Forecasts Using AI

Background
Extreme weather events, such as heat waves, wildfires, hurricanes, and floods, significantly impact agriculture, energy, transportation, and low-resource communities worldwide. While short-term weather forecasting relies on physics-based models, their limitations in long-term forecasting leave communities vulnerable to climate change. Leveraging the increasing availability of meteorological data offers an opportunity to improve sub-seasonal forecasts (15-45+ days) through machine learning, helping communities better prepare and adapt to extreme weather events.
Objective
The project aimed to develop accurate sub-seasonal forecasts of temperature and precipitation by combining physics-based weather forecasting models with machine learning methods. The ultimate goal was to create tools that help communities and industries adapt to the challenges of climate change-induced extreme weather.
Approach
To tackle the challenge, the project followed a systematic approach:
- Data Collection and Preprocessing:
- Collaborated with Climate Change AI (CCAI) to collect meteorological data.
- Augmented datasets with variables like temperature, wind speed, and vapor pressure from NOAA.
- Feature Extraction and Selection:
- Identified key meteorological features influencing weather forecasts.
- Evaluated data relevance for sub-seasonal prediction models.
- Model Development:
- Tested multiple machine learning algorithms, including Random Forests, XGBoost, and Convolutional Neural Networks (CNNs).
- Fine-tuned models for better accuracy and performance.
- Evaluation:
- Assessed models against official NOAA forecasts for one year.
- Compared forecast accuracy metrics for temperature and precipitation.
- Final Presentation:
- Compiled findings into a final report and optional dashboard presentation.
Results and Impact
The project delivered significant outcomes:
- Developed accurate models to predict 14-day mean temperatures, surpassing baseline physics-based forecasts.
- Demonstrated improved long-term prediction reliability through the integration of meteorological data and machine learning techniques.
- Provided actionable insights to enhance disaster planning, agricultural strategies, and energy management in response to climate change.
- Showcased an innovative fusion of AI and climate science, emphasizing the potential for machine learning to drive climate resilience initiatives.
Future Implications
The project’s success highlights the transformative potential of AI in meteorology. Future research could refine models to include additional meteorological variables and address other climate phenomena, paving the way for improved policies and tools for global climate resilience.
This challenge is hosted with our friends at



Become an Omdena Collaborator
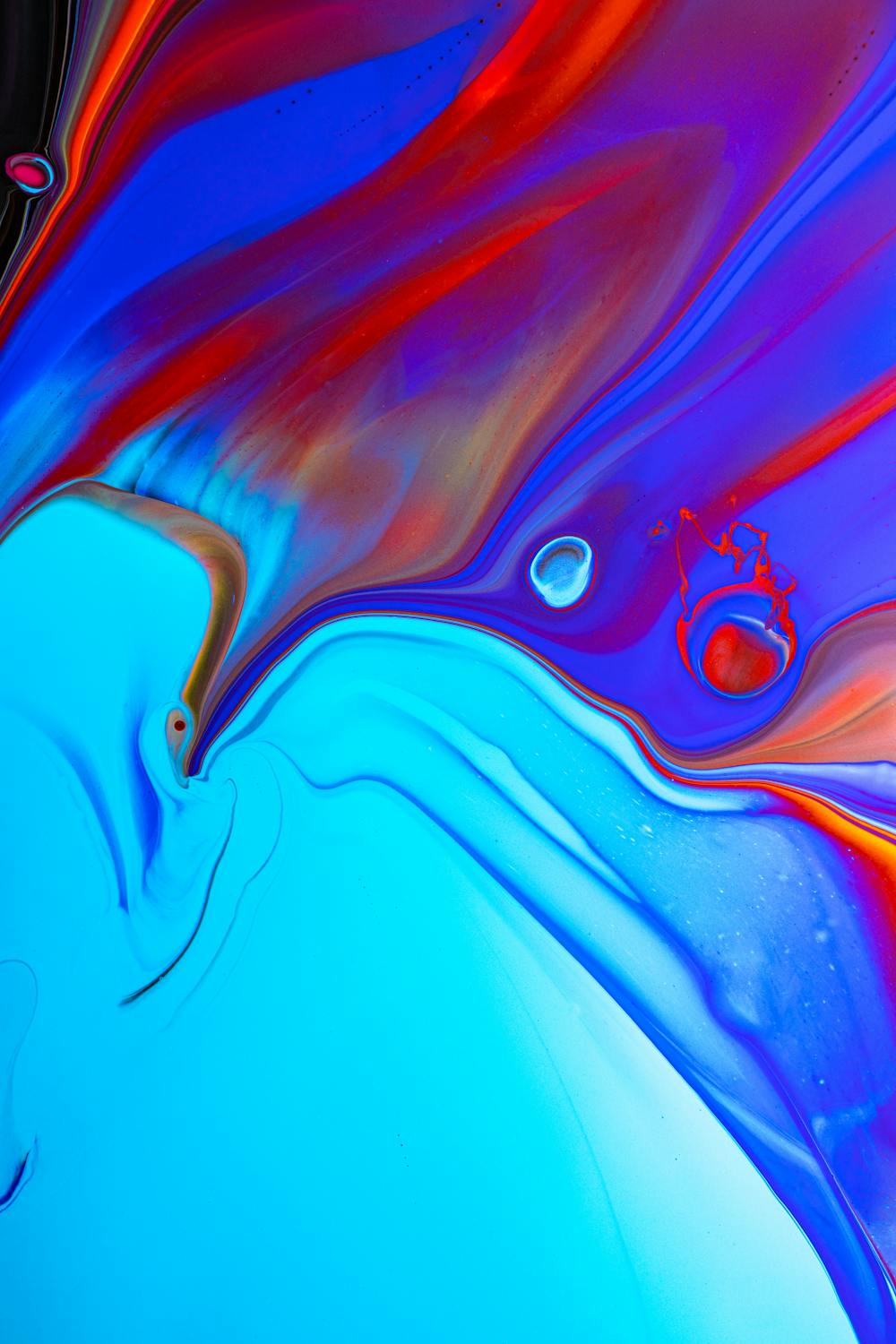