Improving Food Security and Crop Yield in Senegal Through Machine Learning

A challenge initiated to help farmers know where to add water or fertilizer using data such as soil PH, temperature, and moisture levels, combined with other data sources. The data and predictions will also help to decide where to invest, and help strengthen the understanding of crop losses while maximizing revenues and minimizing losses.
The Global Partnership for Sustainable Development Data (GPSDD) seeks to leverage machine learning to help farmers cope with increasingly erratic weather, model the fastest route to markets and mobilities across livelihood zones, and detecting problems in fields with drones and others tools. GPSDD seeked to leverage machine learning to help farmers improve crop yield prediction.
The Problem
At a time where the world needs to produce more with fewer resources, AI could help to transform agriculture worldwide and especially in Senegal. The ability of agricultural equipment to help actors better think, predict, and advise farmers via a variety of AI applications presents Senegal with the potential to achieve food security in the country. Senegal is among those hardest hit by climate change, according to scientists, with populations that depend largely on agriculture losing their livelihoods due to worsening and recurrent floods and droughts.
Using technology, we can now, have better results to know what is going to happen and where in Senegal while promoting a data-driven agricultural system. Data such as soil PH, temperature, and moisture levels, combined with other data sources from ANSD and other stakeholders such as DAPSA, CSE, ANACIM, CSA, could be processed to show exactly when and where farmers should add water or fertilizer. The data will also help to decide where to invest, and help strengthen the understanding of crop losses while maximizing revenues and minimizing losses.
The Results
In these two months, Omdena’s collaborators were able to implement a Deep Learning model that predicts crop yield in Senegal following this schema:
The data used to get crop yield prediction using deep neural networks:
- Remote sensing data downloaded with Google Earth Engine (GEE)
- Ground truth crop yield data: we had yield data collected by IPAR for the production of maize, rice, and millet in 2014
Data engineering and pre-processing were used with the satellite imagery datasets they collected, before feeding them into Convolutional Neural Networks for a Transfer Learning process.
Then the team created an application using open-source satellite images to identify the crops and estimate the yields for any given area.
To read more about the whole process, check the articles below, where the collaborators shared the methodologies used and the essential code.
A demo of the application is attached below
Why an Omdena Project?
Your benefits
Join a thriving AI community in 85 countries
Work with changemakers from around the world
Adress a real-world problem with your skills
Build up your skill-set while setting the stage for a meaningful career
Requirements
Good English
A good/very good grasp in computer science and/or mathematics
Student, (aspiring) data scientist, (senior) ML engineer, data engineer, or domain expert (no need for AI expertise)
Programming experience with C/C++, C#, Java, Python, Javascript or similar
Understanding of ML and Deep learning algorithms
This challenge has been hosted with our friends at
Application Form



Become an Omdena Collaborator
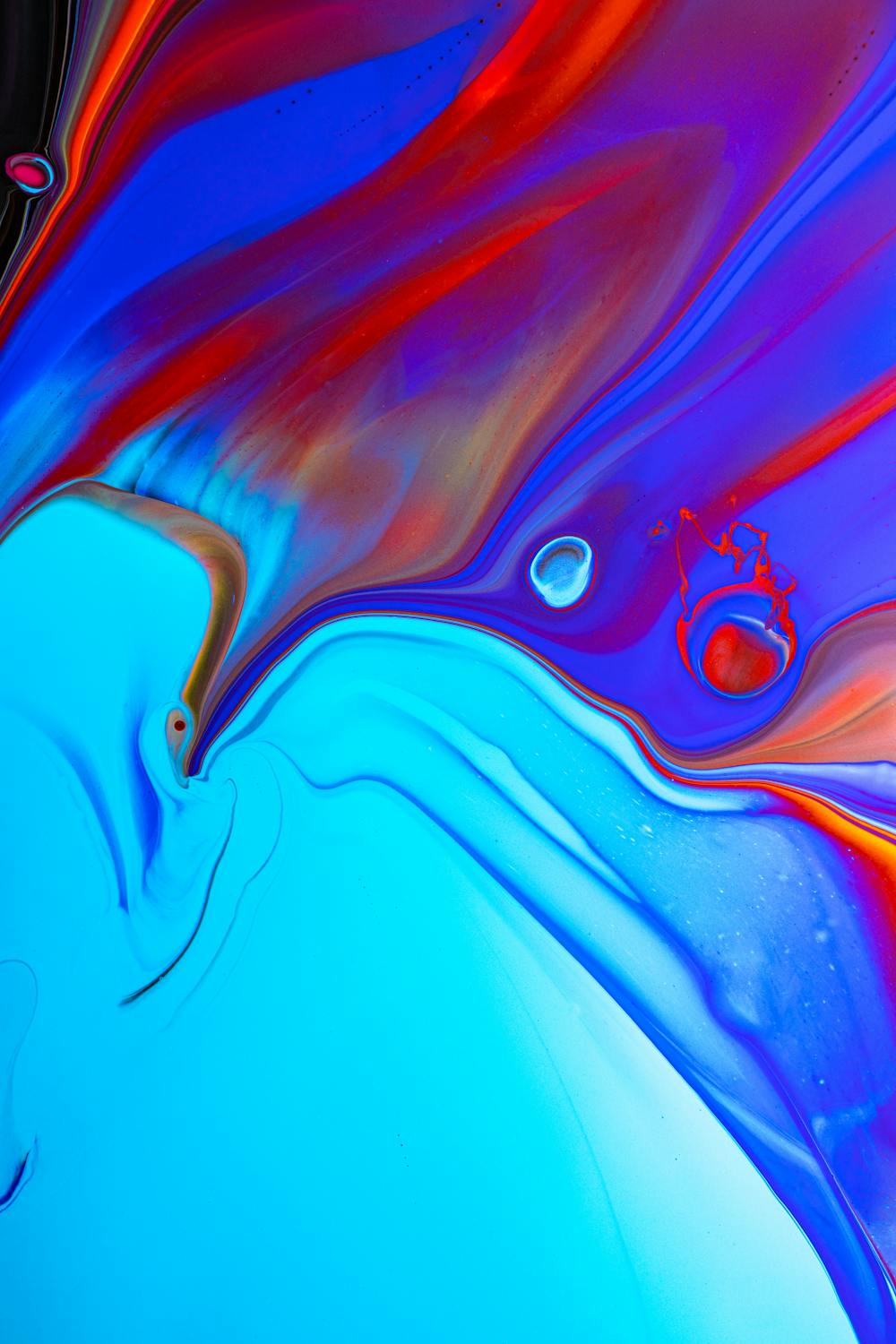