Detecting and Classifying Rooftop Using Neural Networks to Eliminate Energy Waste

Background
Energy companies and sustainability-focused organizations face challenges in accessing reliable customer electricity data, which is essential for identifying efficiency and sustainability opportunities. The lack of this data slows operational decision-making and go-to-market strategies for solutions like solar energy installations, retrofits, and energy consulting.
A Techstars Energy startup sought a digital mapping solution to provide energy assessments for 250,000 facilities across North America, including insights into their kilowatt-hour consumption. One critical indicator of energy consumption is the type and characteristics of facility rooftops.
Objective
The project aimed to develop an AI-powered solution for classifying rooftop types and characteristics to enhance energy consumption understanding. This would support predictive analytics for energy efficiency and sustainability initiatives, enabling faster decision-making for energy sales teams and broader societal impact.
Approach
To address the challenge, Omdena gathered a team of 50 AI changemakers to develop a cutting-edge AI model over two months. The approach involved:
- Data Collection: Access to rooftop images from over 200,000 facilities in North America.
- Model Development: A convolutional neural network (CNN) and edge detection techniques were employed to classify rooftops into five distinct categories:
- Flat and Clear
- Flat and Cluttered (e.g., rooftops with HVAC equipment)
- Light Industrial
- Heavy Industrial
- Existing Solar (rooftops with solar panels)
- Training and Testing: The partner provided labeled image samples to train the CNN. Edge detection was explored to distinguish complex industrial rooftops from simpler ones.
- Technology: Tools included Python-based machine learning frameworks, GIS mapping software, and predictive analytics platforms.
Results and Impact
The project successfully classified rooftop types, providing actionable insights into facility energy intensity. Key outcomes include:
- A scalable AI model capable of analyzing rooftop characteristics for over 200,000 facilities, with plans for global expansion to Canada, Europe, and Asia.
- Enhanced predictive analytics for energy sales teams, accelerating decision-making and improving market targeting.
- Support for energy efficiency and sustainability efforts, reducing time-to-impact for retrofits, solar installations, and other solutions.
- Increased understanding of rooftop complexity, paving the way for additional facility classifications.
This solution strengthens the digital mapping platform, empowering energy companies to quickly identify high-impact facilities, optimize operations, and contribute to a sustainable future.
Future Implications
This AI-driven rooftop detection model has broader applications beyond energy efficiency. Future research could:
- Expand the classification categories to encompass more facility types.
- Integrate rooftop characteristics with real-time energy consumption data for deeper insights.
- Influence policy by demonstrating the importance of accessible electricity data for sustainable development.
- Support global adoption, providing scalable solutions for regions with emerging energy markets.
The project exemplifies how AI can drive transformative impact in sustainability and energy efficiency.



Become an Omdena Collaborator
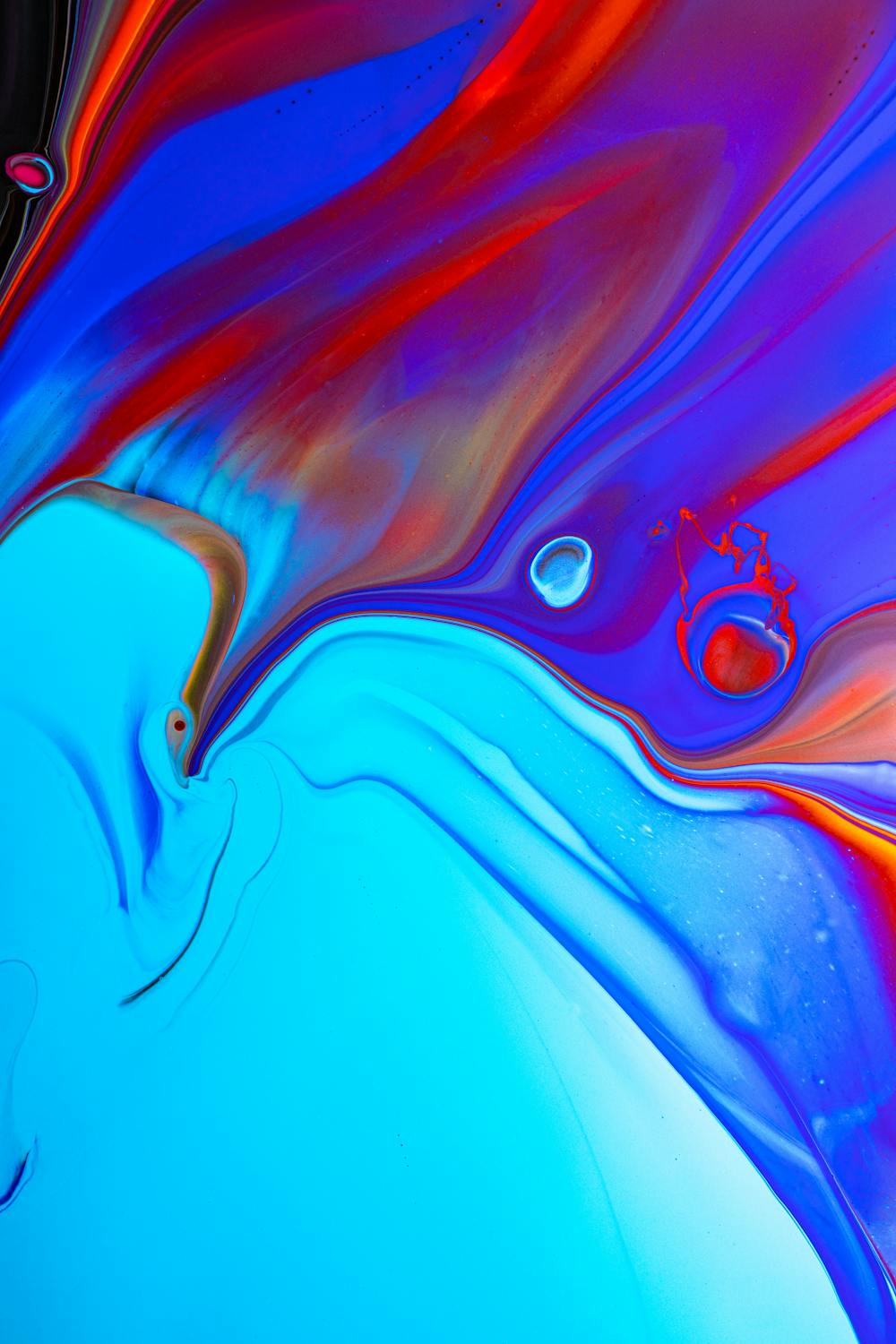