AI in Agriculture: Crop Classification to Fight Hunger in Nepal

Background
Nepal, a country where agriculture sustains 80% of the population, faces significant challenges due to poverty and unstable governance. One-third of Nepal’s population lives below the poverty line, making food security a critical issue. The UN World Food Program (UNWFP) partnered with Omdena to explore how AI could address these challenges. The goal was to leverage machine learning to optimize the growth and monitoring of staple crops like rice and wheat, thereby combating hunger and improving livelihoods.
Objective
The project aimed to develop a machine learning-based crop classification model capable of accurately identifying and tracking staple crops using satellite imagery. This solution sought to assist governmental and non-governmental organizations in making data-driven decisions to allocate resources efficiently, ultimately supporting sustainable agricultural growth.
Approach
The Omdena team, comprising 32 collaborators from 18 countries, faced challenges such as limited access to high-quality data. To overcome these issues, the team:
- Aggregated diverse datasets, including Sentinel satellite images from the Copernicus program and the EuroSAT dataset.
- Leveraged advanced deep learning techniques, such as super-resolution, to enhance data quality.
- Built a machine learning model with 89% accuracy for crop identification.
- Created a seven-point recommendation guide for developing satellite imagery datasets tailored for agricultural applications.
Results and Impact
The project successfully delivered a high-accuracy crop classification model, demonstrating the potential of AI in agriculture. The model serves as a prototype for the UNWFP, showcasing how open-source data and machine learning can improve agricultural decision-making. The solution enables effective resource allocation to boost the growth of essential crops like rice and wheat, contributing to food security in Nepal.
Future Implications
This project sets a precedent for the use of AI in addressing agricultural challenges in developing countries. The findings have the potential to guide future policies and foster further research on the use of satellite imagery and machine learning to improve crop management and food security. By building confidence in AI-driven solutions, this initiative paves the way for broader adoption of similar technologies in other regions.
This project has been hosted with our friends at



Become an Omdena Collaborator
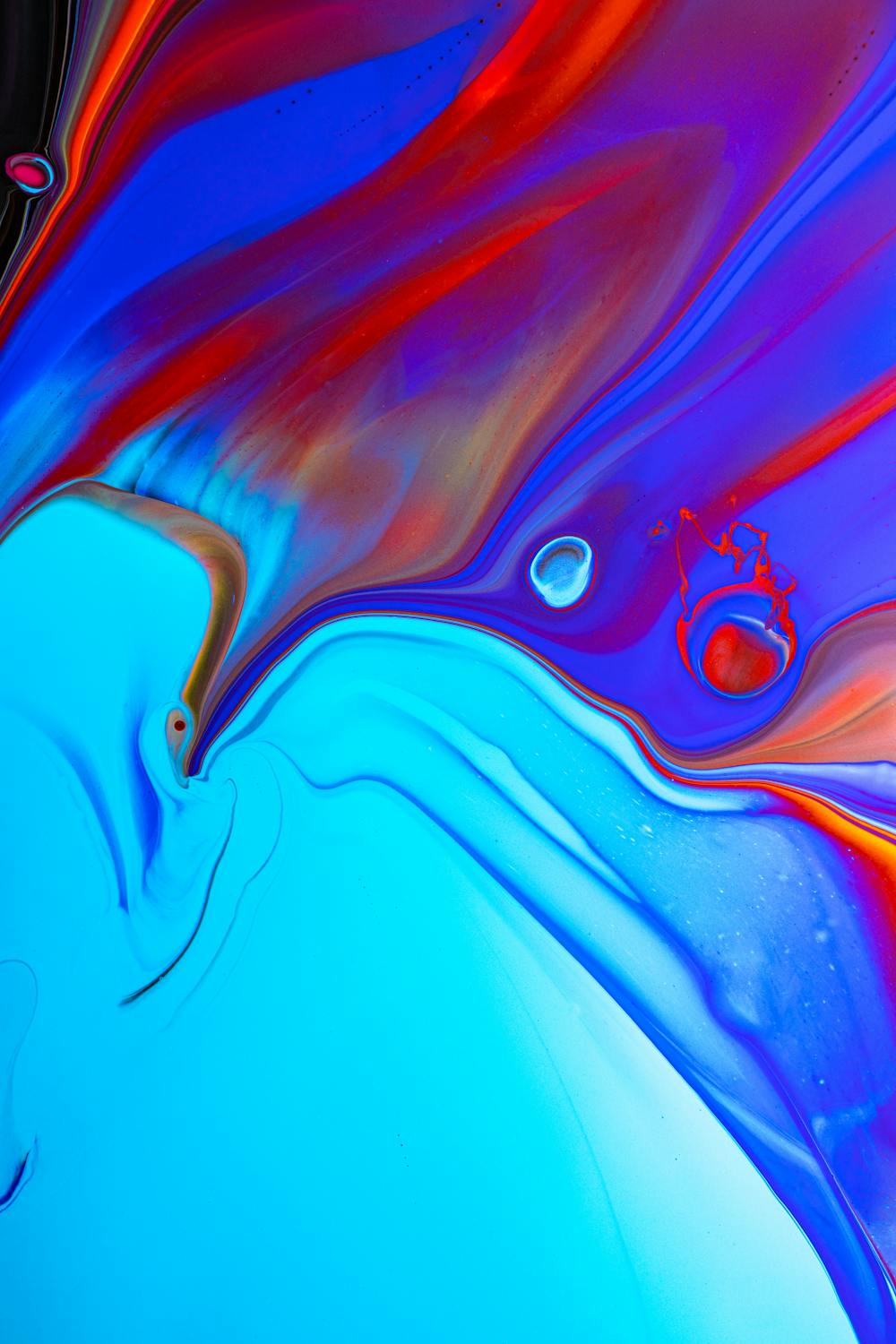