NLP Empowered Climate Data Dashboard: Visualizing Climate Change Impacts and Mitigating Nature-Based Solutions

Background
The World Resources Institute (WRI) is spearheading efforts to explore the potential of Nature-Based Solutions (NbS) in mitigating the impacts of climate change. NbS, such as forest and landscape restoration, are critical for addressing environmental challenges. Despite the increasing focus on these approaches through initiatives like The African Forest Landscape Restoration Initiative( AFR100), Initiative 20×20, and Cities4Forests, the adoption and integration of NbS into climate adaptation strategies remain limited and under-researched. These gaps hinder effective responses to climate hazards, necessitating innovative solutions to enhance implementation and awareness.
Objective
The project aimed to analyze the approaches of various NbS coalitions and their member platforms in addressing climate risks. Key goals included:
- Assessing how NbS platforms address climate hazards.
- Identifying types of NbS solutions being adapted.
- Uncovering barriers and opportunities for successful NbS adoption.
- Developing tools to visualize and analyze climate impacts and NbS interventions effectively.
Approach
The Omdena team deployed advanced technologies such as machine learning and Natural Language Processing (NLP). Key methodologies included:
- Website Analysis: Examining coalition and member websites for climate adaptation strategies.
- Data Visualization: Creating choropleth maps to represent annual changes in climate and landscape parameters, including land type cover, temperature, and soil moisture.
- Deforestation Evaluation: Analyzing deforestation trends to inform NbS strategies.
- Heat Maps: Measuring relevance between climate risks and NbS interventions.
- Knowledge-Based Systems: Employing NLP to develop a Question & Answer system for extracting information from text and PDFs.
- Recommendation System: Implementing content-based filtering to suggest relevant NbS strategies using collaborative insights.
Results and Impact
The project delivered an interactive dashboard that visualizes the impacts of climate change and highlights NbS as mitigation tools. Key outcomes included:
- Enhanced Visualization: Choropleth and heat maps providing actionable insights into climate risk adaptation.
- Knowledge Access: An NLP-powered Q&A system that enables stakeholders to explore NbS strategies comprehensively.
- Recommendations: A recommendation engine suggesting tailored NbS interventions for specific climate risks.
These tools empower policymakers, researchers, and organizations to make informed decisions, fostering effective adoption of NbS to combat climate change.
Future Implications
The findings and tools developed in this project can shape the future of Nature-Based Solutions by:
- Informing sustainability policies and climate adaptation strategies.
- Encouraging ESG (Environmental, Social, and Governance) integration by showcasing the tangible benefits of NbS.
- Guiding further research on climate resilience and ecosystem restoration.
- Supporting the scalability of NbS initiatives across regions and sectors.
By enhancing the understanding and adoption of NbS, this project paves the way for actionable strategies to combat climate change.
This challenge is hosted with our friends at



Become an Omdena Collaborator
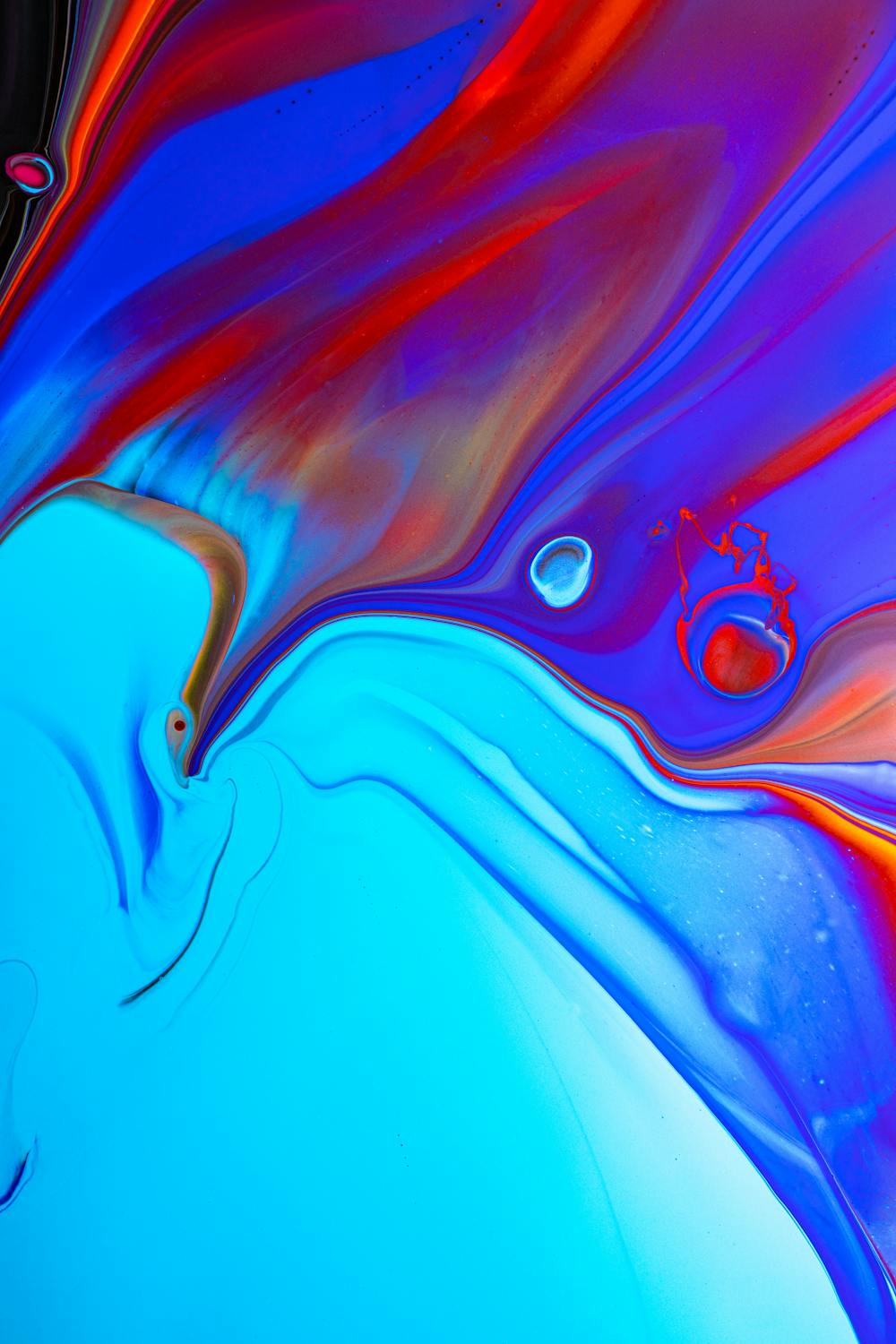