Weed Detection Using Computer Vision to Reduce Environmental Footprint

Background
The agricultural industry faces a critical challenge in managing weed growth without relying on chemical herbicides, which harm soil health, groundwater quality, and biodiversity. Conventional weed control methods often lead to increased environmental pollution and make organic food production expensive and less accessible. To address this, the impact-driven startup Weedbot has developed innovative laser weeding machinery that uses advanced weed detection technology. Omdena contributed by building machine learning models to advance pesticide-free farming practices.
Objective
The project’s primary goal was to enable precise, efficient, and environmentally sustainable weed control by developing machine learning models capable of:
- Localizing and identifying plants.
- Distinguishing crops from weeds with high accuracy.
- Facilitating the implementation of laser weeding technology to reduce herbicide use and promote sustainable agriculture.
Approach
Omdena and Weedbot collaborated to design and train high-speed neural networks for weed detection. The project included the following steps:
- Data Collection: Captured plant images with a working area of 200×200 mm, focusing on carrot seedlings.
- Model Development: Developed a plant image recognition neural network tailored for real-time weed segmentation.
- Tools and Techniques: Utilized advanced machine learning frameworks and image processing tools such as PlantCV to enhance accuracy.
- Performance Optimization: Achieved recognition precision of 1–2 mm with a processing speed of 12 ms per image or faster.
The model achieved up to 10% allowable false positives while detecting 100–110% of crop polygons. This ensures all crops are accurately recognized, enabling precise weed removal without harming the desired plants.
Results and Impact
The project delivered a robust AI-powered weed detection system that:
- Precision and Speed: Achieves 1–2mm precision with rapid image processing.
- Scalability: Supports weed segmentation for a wide range of crops, starting with carrots.
- Environmental Benefits: Reduces reliance on chemical herbicides, mitigating soil and groundwater contamination.
- Pesticide-Free Farming: Paves the way for affordable organic food production, encouraging healthier lifestyles and sustainable agriculture practices.
These advancements make laser weeding a practical alternative to chemical-based solutions, contributing to a cleaner and greener agricultural ecosystem.
Sample image with annotated carrots:
Insight from Weedbot CEO on the project
Future Implications
The success of this project demonstrates the potential of AI-driven weed detection and laser technology to revolutionize agriculture. Future applications could include:
- Wider Crop Coverage: Expanding weed detection models to handle various crops.
- Policy Influence: Advocating for reduced herbicide use in agricultural practices.
- Technological Integration: Further integration of AI and robotics for fully automated pesticide-free farming.
- Research Expansion: Leveraging data insights to refine weed detection models and improve performance.
By advancing these technologies, we can work towards a sustainable food system that prioritizes health, affordability, and environmental stewardship.
This challenge is hosted with our friends at



Become an Omdena Collaborator
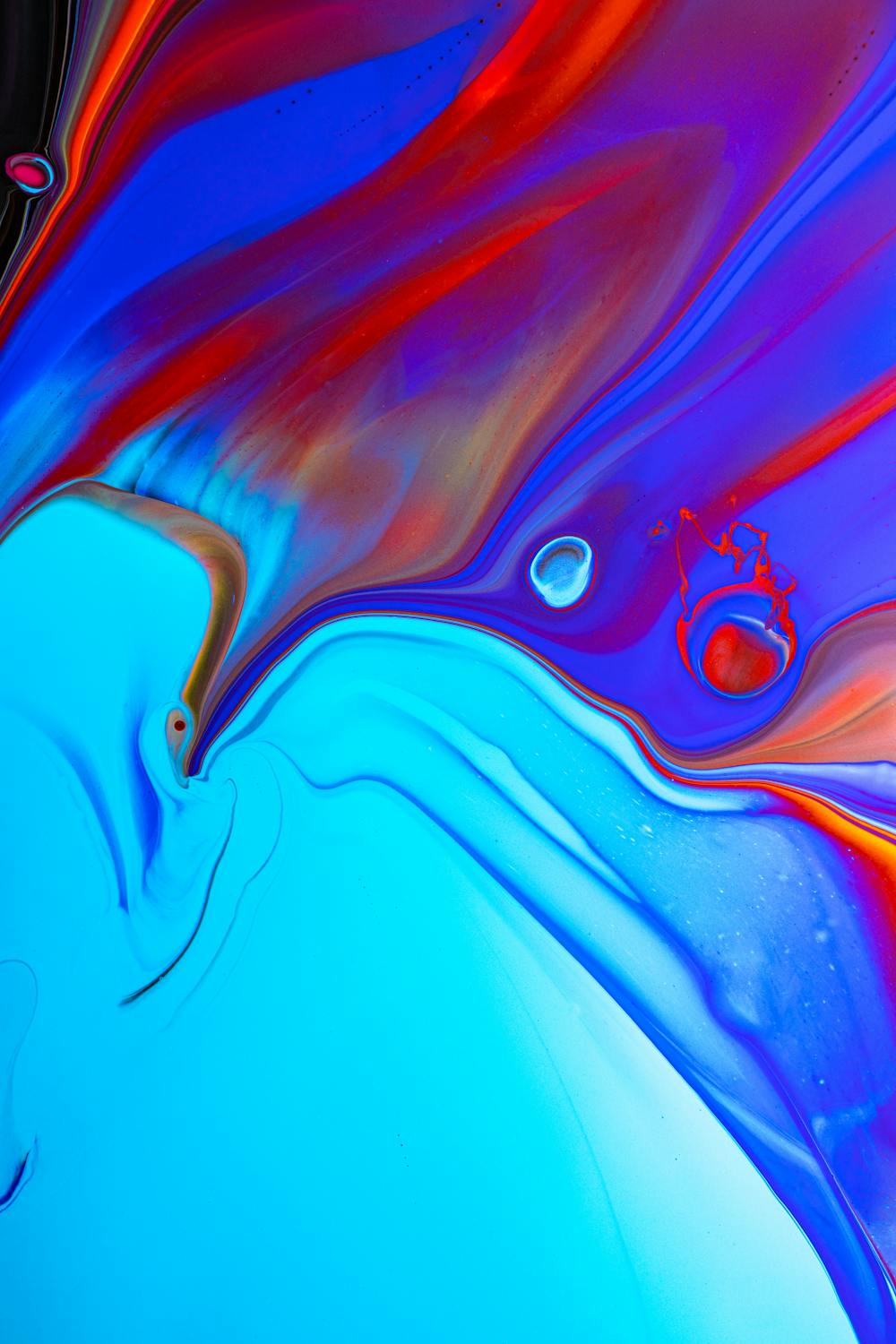