Improving Natural Disaster and Water Resource Management using Natural Language Processing

Background
WEO, a startup focused on sustainability, sought to improve water resource management (WRM) by leveraging space-based data. Rapid urbanization, population growth, dietary shifts, and climate uncertainties make effective WRM vital for ensuring adequate water quality and quantity for drinking, sanitation, food production, energy, and ecosystems. The challenge was to create innovative solutions for WRM that address natural calamities like floods and droughts while promoting environmental balance.
Objective
The project aimed to develop a tool that allows WEO to query critical mission-specific data from the internet. This tool would enable better understanding and management of water resources by gathering and analyzing information on natural disasters and urban climate conditions.
Approach
Over eight weeks, a team of 50 changemakers collaborated to build a comprehensive data solution. The tool allows users to define topics, areas of interest, and timeframes to extract relevant information. Key methods and tools included:
- Data Analysis: Extracting and analyzing data from internet sources.
- Query Customization: Users could specify parameters such as topic, location, and timeframe.
- Data Storage and Evaluation: Developing mechanisms to store and statistically analyze retrieved data.
Example query topics included:
- Flood Evaluation: Gathering data on rainfall, water levels, public reports, images, and official flood evaluation platforms.
- Drought Evaluation: Collecting data on plant health, water level changes, and irrigation-related measures.
- Landcover Mapping: Obtaining information on crop planning, green roofs, and pavement installations.
- Urban Climate: Retrieving data on temperature sensors, HVAC system performance, and public perceptions of urban heat.
Results and Impact
The project delivered a powerful, multifaceted tool that supports advanced WRM and disaster evaluation by providing:
- A flexible query system for mission-critical data.
- Effective storage and statistical analysis capabilities.
- Practical applications in flood and drought evaluations, landcover mapping, and urban climate monitoring.
This innovation accelerates the development of WRM solutions, helping to mitigate risks from natural disasters and ensure sustainable water use.
Future Implications
The findings from this project could shape future WRM policies, drive further AI-driven research, and foster advancements in space-based data applications. By scaling the tool’s capabilities, governments, industries, and communities can better prepare for and adapt to water-related challenges in a changing climate.
This challenge has been hosted with our friends at



Become an Omdena Collaborator
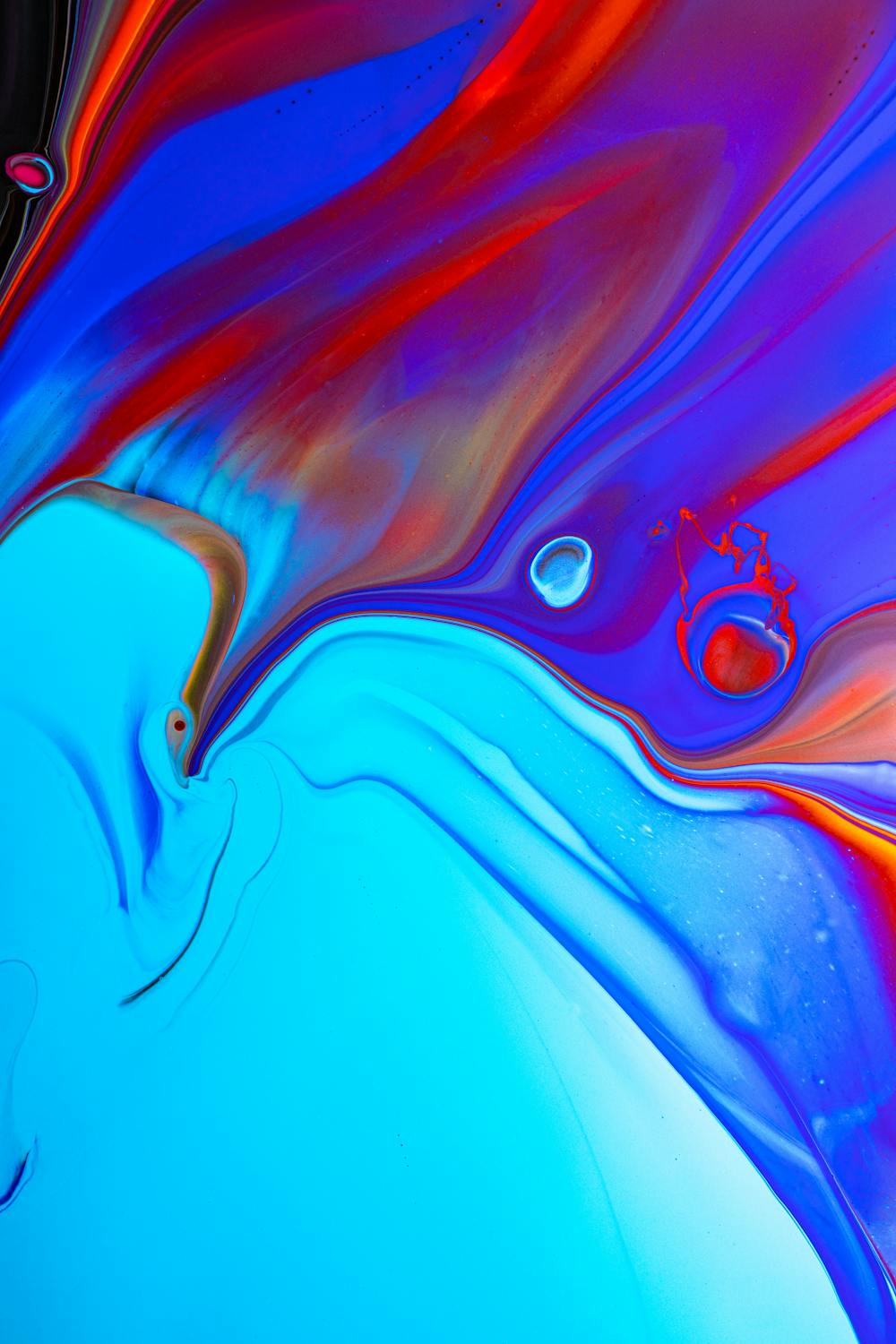