Increasing Solar Energy Adoption Through Roof Detection

Solar AI, a Singapore based startup incubated as a part of ENGIE Factory, collaborated with Omdena, to hyper-scale the deployment of distributed solar and the transition towards 100% renewables by modernizing the way rooftop solar is sold.
The problem
The rooftop solar assessment process can be time-consuming and expensive, taking anywhere between 1 hour to 2 full days to calculate the solar potential of each rooftop. In the solar industry, this has resulted in the cost of sales taking up to 30–40% of total project costs, significantly worsening the unit economics of solar projects.
By automating this process, Solar AI aims to drastically reduce the cost of this process and make this information easily available for both building owners as well as solar energy companies.
The project outcomes
The data
Even the most technically advanced algorithms cannot address or solve a problem without the right data. Having access to data is quite valuable, but having access to data with a learnable structure is the biggest competitive advantage nowadays. Our team of collaborators annotated thousands of rooftops. The consistent determination of the annotators resulted in a perfectly labeled dataset for Supervised Learning algorithms.
The machine learning models
The major task was to detect rooftops in a given image using Computer Vision models. We also had to determine their type/structure such as Flat-roof, Hip-roof, Shed-roof, or any other. Hence, this became an Instance Segmentation problem. The team tried out a number of models such as Mask R-CNN, YOLACT (You Only Look At CoefficienTs), Dectectron2, and more. After training on different batches of annotations as they were delivered, we kept seeing improvement in results. Eventually, the best-performing model was selected to go ahead with other tasks.
Now that we had the bounding boxes and mask contours of various rooftops, trapped properly in a data frame, we were ready to start the analysis of individual rooftops. After extracting and zooming into masks of each detected roof, we needed the following attributes:
- Obstacle Detection
- Area of the roof (excluding obstacles)
- Material of the roof
- Detecting faces of Hip/Shed roof
- Orientation of individual slopes
For the calculation of a rooftop’s effective area, the area occupied by obstacles has to be subtracted from the whole. So that gives rise to the task of identifying obstacles.
After merging everything into an automated pipeline and many rounds of reviews, evaluation, fixing bugs, and testing — our software was ready to be delivered.
The project falls under the UN´s Sustainable Development Goal 7, which is to ensure access to affordable, reliable, sustainable, and modern energy for all.
Project completed!
Your benefits
Working with world-class mentors and domain experts to acquire real-world experience
Making international friends in a fast-growing supportive community of collaborators
Boosting your technical skills, problem-solving capabilities, and collaboration skills
Building your personal brand and publishing your own articles on our website and blog
Receiving certificates of participation and references to accelerate your career
Requirements
Good English
A good/very good grasp in computer science and/or mathematics
Student, (aspiring) data scientist, AI engineer, data engineer, domain expert (no need for AI expertise)
Programming experience with C/C++, C#, Java, Python, Javascript or similar
Understanding of ML and Deep learning algorithms
This project has been hosted with our friends at
Application Form
Related Projects



Become an Omdena Collaborator
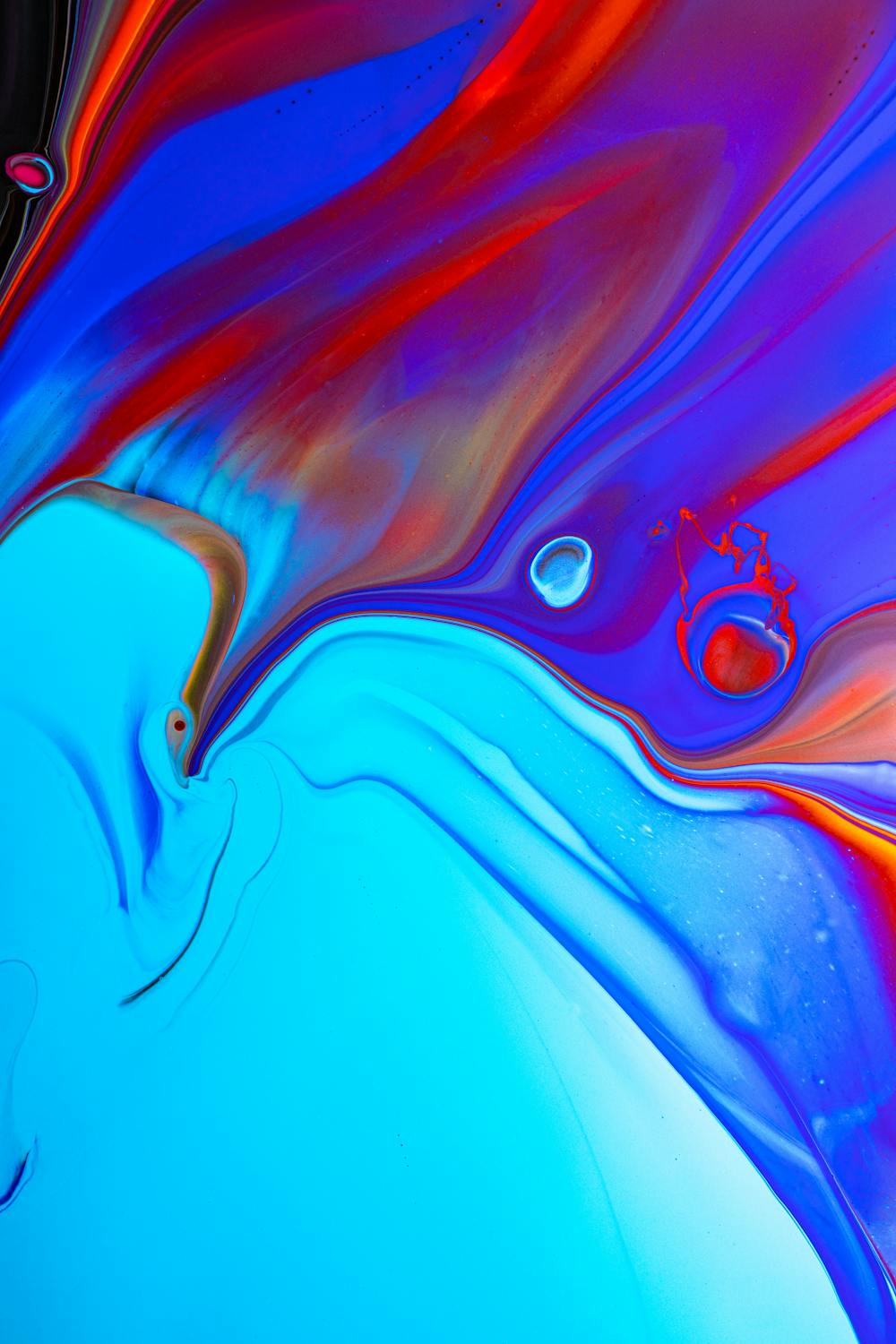