AI for Road Safety: Preventing Road Crashes Through Machine Learning

A car accident with a damaged automobile on the road.
Background
Road crashes are the leading cause of death for young people aged 5 to 29 worldwide, with a projected 500 million individuals facing life-altering injuries by 2030. This tragic trend demands urgent solutions. The International Road Assessment Programme (iRAP), a charitable organization, aims to mitigate these risks by transforming dangerous roads into safe pathways. iRAP collaborates globally to assess crash risks and enhance road safety standards, yet the challenge persists in efficiently mapping and improving road infrastructure across over 100 million km worldwide.
Objective
The AI for Road Safety project aims to achieve three critical goals under the iRAP initiative:
- Generate iRAP Risk Maps using geo-located crash data.
- Map safety performance and assign Star Ratings to roads by analyzing road attributes, traffic flow, and speed data.
- Develop repeatable key performance indicators for ongoing road infrastructure assessment and improvement.
Approach
The project brought together over 30 machine learning engineers and subject matter experts to advance iRAP’s vision of “a world free of high-risk roads.” Key methodologies included:
- Data Sourcing and Analysis: Leveraged geo-located crash data, road attribute data, and traffic flow statistics from iRAP’s extensive database, which includes over 1 million km of road data.
- AI-Powered Assessments: Developed machine learning models to automate critical tasks, such as extracting vehicle count data from satellite imagery for traffic flow analysis.
- Risk Mapping and Performance Indicators: Used AI to produce iRAP Risk Maps and Star Ratings, providing actionable insights for road safety improvements.
Results and Impact
The project contributed to the United Nations Sustainable Development Goal of halving road deaths and injuries by 2030. By enhancing road assessments with AI, the initiative:
- Supported the development of safer infrastructure, ensuring all new roads achieve at least a 3-star rating.
- Aimed to save 450,000 lives annually and prevent over 100 million injuries.
- Generated significant economic savings, with an estimated $8 return for every $1 invested.
- Empowered road safety stakeholders in over 100 countries with AI-driven insights and tools.
Future Implications
The findings highlight the potential for AI to revolutionize global road safety efforts. By enabling more frequent, accurate, and cost-effective road assessments, this approach:
- Lays the groundwork for smarter infrastructure investments and public safety policies.
- Demonstrates how AI can scale to address large-scale challenges, paving the way for further innovations in road safety and urban planning.
- Encourages international collaboration to achieve safer roads and sustainable development goals.
AI for Road Safety is a transformative step towards reducing road trauma, protecting lives, and building a safer future for all.
This challenge has been hosted with our friends at



Become an Omdena Collaborator
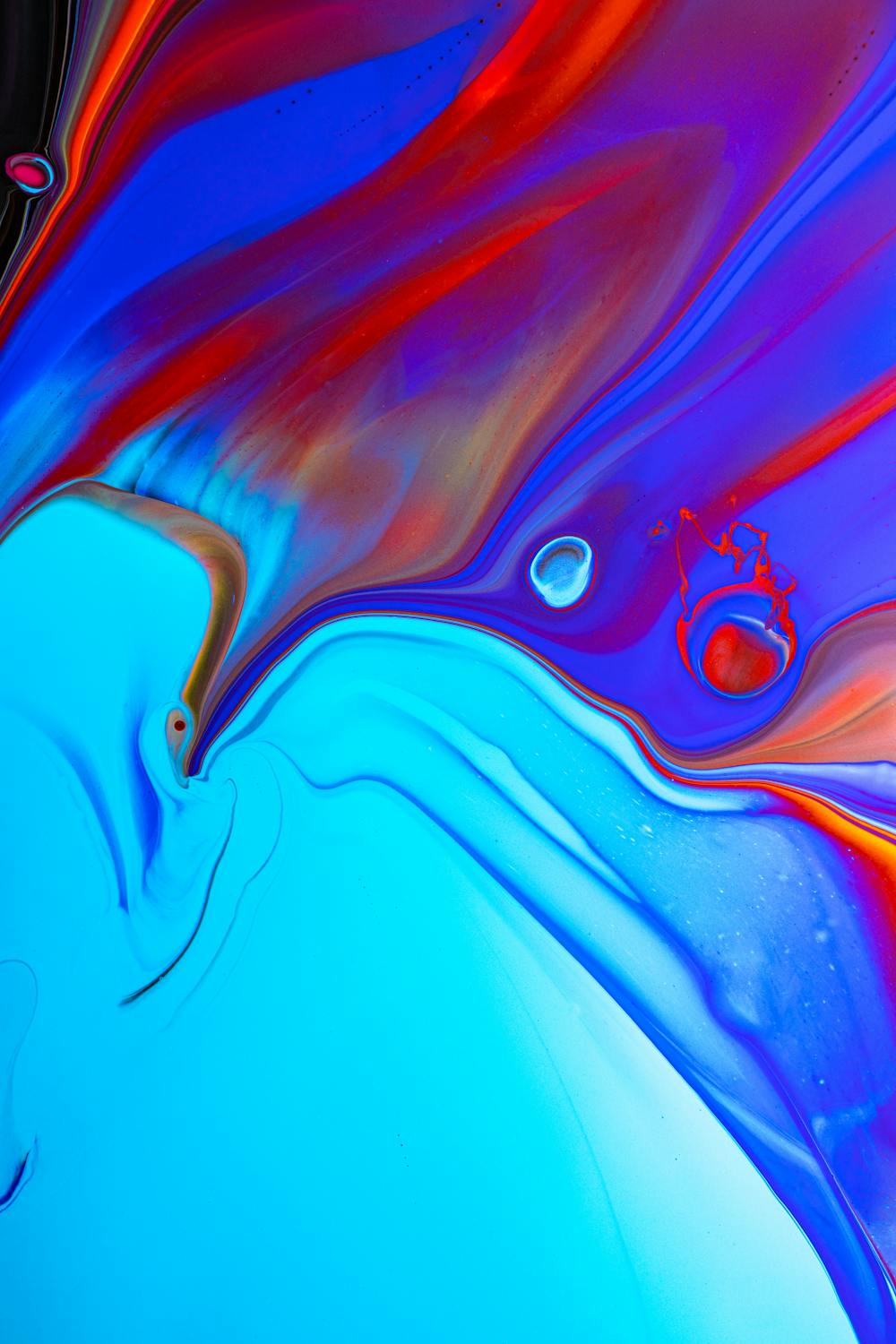