Measuring Soil Organic Carbon with AI for Regenerative Farming Practices

Background
The EU’s commitment to achieving carbon neutrality by 2050 emphasizes the importance of reducing emissions across all sectors, including agriculture. Agriculture is a significant contributor to carbon emissions, yet it holds tremendous potential for carbon sequestration through practices that increase soil organic carbon (SOC). However, barriers such as the high cost of SOC monitoring, lack of knowledge about regenerative farming practices, and limited financial resources hinder the transition to sustainable models. Additionally, companies face challenges ensuring accurate carbon offset measurements to comply with legal and social responsibilities.
Objective
The project aimed to:
- Develop a data-driven tool to measure and predict soil organic carbon (SOC).
- Provide actionable insights for farmers to transition toward regenerative agriculture.
- Support companies in certifying carbon offsets and aligning their operations with Sustainable Development Goals (SDGs).
Approach
To address these challenges, the team implemented several steps:
- Created a data visualization dashboard integrating various datasets and predictive models.
- Utilized farming data from the project partner, SigPac, WISE-3, and additional resources like the ICGC website and LUCAS topsoil dataset.
- Applied machine learning models to analyze and predict SOC levels across different land types.
- Focused on excluding non-agricultural areas (e.g., grasslands, shrublands, forests) to refine SOC predictions for farmland.
Results and Impact
The project delivered a user-friendly online data visualization tool to:
- Enabled the analysis and comparison of regenerative farming practices and their impact on SOC.
- Highlighted areas with the highest SOC (e.g., grasslands, shrublands, forests) and their exclusion for accurate agricultural SOC measurement.
- Identified regional variations in biomass, such as a decrease in 2021, except in the Western Region of Catalunya.
Key Impact:
- Enhanced understanding of SOC trends using historical and geospatial data.
- Provided a pathway for farmers to adopt regenerative practices that improve soil quality and sequester more carbon.
- Supported businesses in certifying carbon offsets to meet environmental and regulatory standards.
Future Implications
The findings have significant implications for agriculture and climate change mitigation:
- Policy Development: Data-driven insights can guide policies to incentivize regenerative farming practices.
- Regenerative Farming: Scalable models and tools will help farmers adopt sustainable practices effectively.
- Future Research: The dashboard’s framework can be expanded to include global datasets, enabling more comprehensive SOC predictions.
- Climate Action: Accurate SOC monitoring can play a pivotal role in achieving carbon neutrality goals and enhancing soil health.
This project exemplifies the power of AI in addressing complex environmental challenges and underscores the need for innovative approaches to sustainable agriculture.
This challenge has been hosted with our friends at



Become an Omdena Collaborator
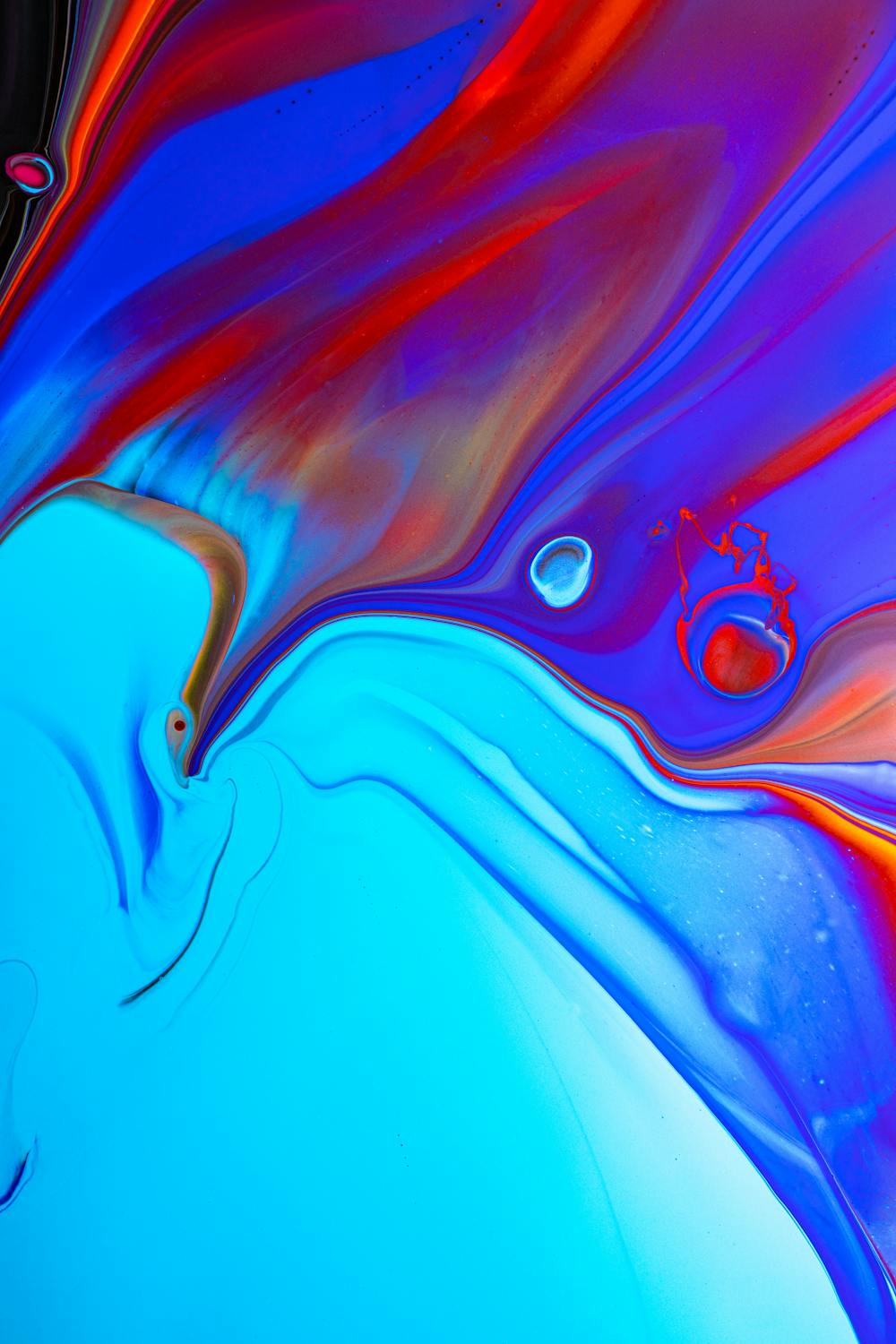