Analyzing Citizen’s Sentiment on the Energy Transition Using Natural Language Processing

Background
The global energy transition represents a massive shift from reliance on climate-damaging fuels to sustainable energy systems. While governments and corporations play pivotal roles, individuals’ perceptions and actions are equally critical. Omdena collaborated with the World Energy Council to use artificial intelligence (AI) to understand public attitudes toward the energy transition, shedding light on its societal challenges and opportunities.
Objective
The project aimed to leverage AI-driven natural language processing (NLP) to analyze public sentiments about the energy transition across diverse regions. Key objectives included identifying societal concerns, analyzing public perceptions of renewable energy costs, and providing insights to guide clean and socially equitable energy policies.
Approach
The team involved a systematic approach to tackle the challenges of analyzing global sentiments on the energy transition:
1. Development of Advanced AI Models:
- Built AI models specializing in Natural Language Processing (NLP) to process and understand human language effectively.
- Designed models capable of analyzing vast datasets and extracting relevant insights.
2. Data Collection:
- Gathered extensive data from multiple sources, including:
- Social media platforms like Twitter to capture public conversations.
- News articles discussing energy-related topics to understand broader societal narratives.
- Focused on gathering diverse data from over 20 countries to ensure global representation.
3. Categorization of Conversations:
- Used AI models to classify public discussions into distinct categories such as:
- Concerns about renewable energy costs.
- Policy-related challenges.
- Technological advancements and barriers.
4. Regional Sentiment Analysis:
- Processed country-specific data to uncover diverse regional perspectives and sentiments.
- Highlighted differences such as:
- Technology concerns dominating in Brazil and France.
- Policy-focused conversations being prevalent in Nigeria.
5. Key Tools and Techniques:
- Social Media Scraping: Automated collection of tweets and posts from platforms to identify trends.
- Data Visualization: Created graphical representations of key findings to make insights accessible.
- Sentiment Analysis: Employed NLP techniques to gauge public attitudes, ranging from support to skepticism.
6. Collaborator Contributions:
- Applied specialized models to filter and visualize sentiment trends in regions like the USA, UK, Nigeria, and India.
- Generated charts and visual data to highlight the most discussed energy transition topics in each region.
Results and Impact
The AI models uncovered critical insights into public concerns, such as:
- In Brazil and France, technology-related challenges dominated renewable energy cost complaints.
- In Nigeria, policy issues were the primary focus.
- Visualizations of tweets in countries like the USA, UK, and India highlighted regional differences in public sentiment.
This work demonstrated the potential of AI to inform sustainable energy policies by analyzing vast public discourse data. By revealing the diverse concerns and priorities of individuals worldwide, the project provided actionable insights to guide an inclusive and equitable energy transition.
Future Implications
The findings underscore the need for tailored communication and policy strategies in the energy transition, reflecting regional challenges and priorities. Future work could expand to analyze more localized data, refine NLP models for greater accuracy, and explore real-time sentiment tracking. These insights could significantly influence future policies and research, ensuring a just and effective global shift to sustainable energy.
This project is hosted with our friends at



Become an Omdena Collaborator
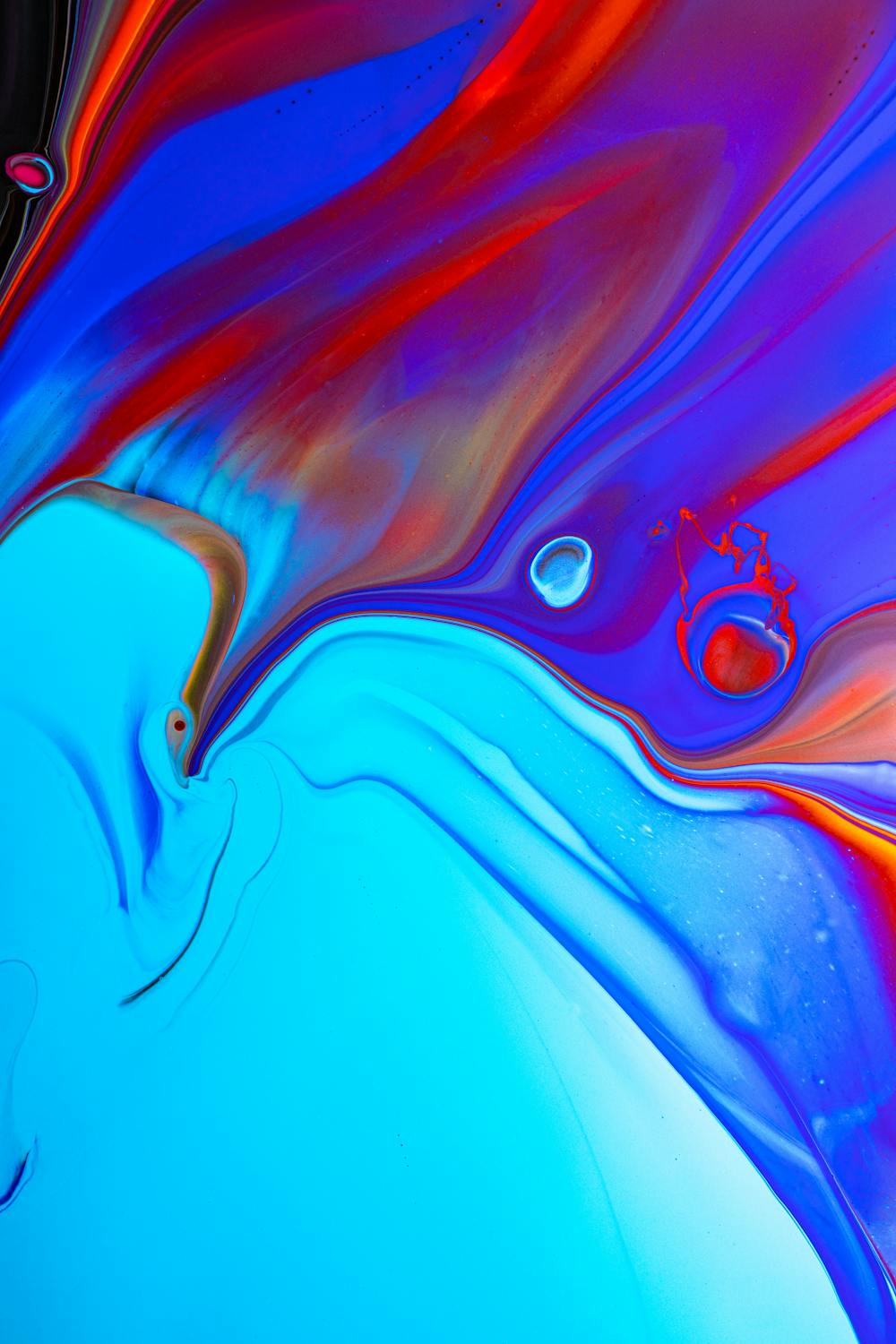