Predicting Cargo Claims Using Computer Vision and Machine Learning

Background
A cargo claim is a demand for monetary compensation due to financial losses caused by a breach of contract during carriage or a carrier’s failure to meet obligations. However, carriers are often exempt from liability under certain legal or contractual circumstances. Inefficiencies in addressing cargo claims reduce profitability and have negative societal and environmental consequences.
The Omdena challenge aimed to tackle this by developing an application to identify safe delivery conditions, including analyzing container-stuffing patterns, safe storage temperatures, and damage assessments through phone images.
Objective
The project focused on:
- Developing a phone application to assess delivery conditions of cargo.
- Using AI to predict damage likelihood and settlement outcomes.
- Overcoming challenges in object detection caused by obstructions or overlapping objects.
- Determining the impact of storage time and temperature on cargo safety.
- Scraping existing case laws to identify patterns for legal claims.
Approach
A global team of 50 AI changemakers collaborated over two months to build a solution using the following methods:
- Data Sources: Images of container boxes, case law data, and historical records of cargo claims.
- Tools Used: AI-powered image recognition, object detection algorithms, and legal text mining.
- Techniques:
- Randomized selection of boxes to inspect through a mobile app.
- Analyzing temperature sensitivity and prolonged storage effects on cargo.
- Image analysis to detect damage and classify conditions under obstacles.
- Comparing existing case law to measure similarities and determine likely settlements.
Results and Impact
- Developed a phone application capable of assessing box conditions in real time.
- Enhanced cargo claims prediction with 72% of similar cases settled at 60% of the claim amount.
- Overcame object detection challenges, such as recognizing cropped or obscured boxes in images.
- Provided actionable insights for carriers to reduce inefficiencies, improving profitability and sustainability.
The project contributes to smarter, data-driven decisions in the logistics industry, optimizing resources and reducing waste.
Future Implications
These findings pave the way for:
- Enhanced automation of claims processing in the logistics industry.
- Further research into integrating AI with IoT for real-time cargo monitoring.
- Improved legal frameworks for faster and fairer resolution of cargo claims.
- Expanding the solution’s application to broader areas, such as cold-chain logistics and other temperature-sensitive industries.
By harnessing AI, this project sets a new benchmark in reducing inefficiencies in cargo handling and claims management.



Become an Omdena Collaborator
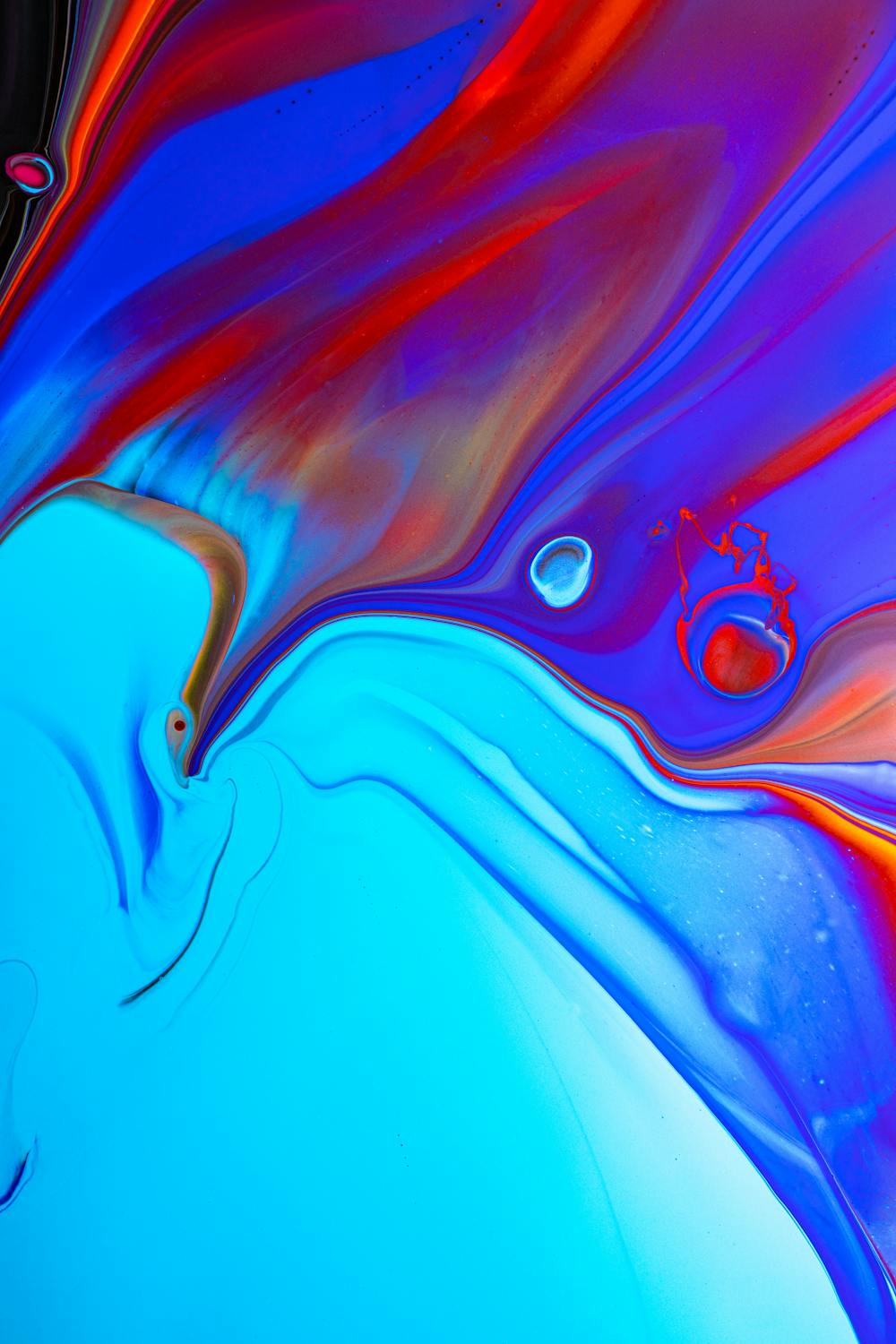