Natural Language Processing (NLP) Fundamentals

For whom is this course?
This course provides a comprehensive introduction to Natural Language Processing (NLP), a branch of artificial intelligence that focuses on the interaction between computers and human language. This course is designed for beginners and professionals seeking to delve into the field of NLP. It is suitable for software engineers, data scientists, linguists, researchers, and anyone interested in leveraging NLP techniques for various applications.
What will you learn?
Participants will explore fundamental concepts, techniques, and applications of NLP, gaining practical skills essential for understanding and working with textual data.
Prerequisites
- Basic programming knowledge in Python is recommended.
- Familiarity with data structures, algorithms, and machine learning concepts would be beneficial but not required.
Syllabus
1. Introduction to NLP
- Overview of NLP applications and challenges
- Introduction to text data and its characteristics
2. Text Preprocessing
- Tokenization
- Stopword removal
- Stemming and lemmatization
3. Language Models
- Bag-of-Words model
- Term Frequency-Inverse Document Frequency (TF-IDF)
- Word Embeddings (Word2Vec, GloVe)
4. Syntax and Semantics
- Part-of-Speech Tagging
- Syntax parsing
- Named Entity Recognition
5. Sentiment Analysis
- Understanding sentiment analysis tasks
- Sentiment lexicons and rule-based approaches
- Machine learning approaches for sentiment analysis
6. Text Classification
- Supervised learning for text classification
- Feature extraction techniques
- Classification algorithms (Naive Bayes, Support Vector Machines, Neural Networks)
7. Topic Modeling
- Latent Dirichlet Allocation (LDA)
- Non-negative Matrix Factorization (NMF)
- Applications of topic modeling
8. Sequence-to-Sequence Models
- Introduction to sequence-to-sequence architecture
- Applications in machine translation, summarization, and question answering
9. Advanced NLP Techniques
- Named Entity Recognition (NER) using deep learning models
- Dependency parsing
- Coreference resolution
10. NLP Applications and Future Trends
- Overview of real-world NLP applications
- Ethical Considerations in NLP
- Future trends and directions in NLP research and development
Instructors
Course Info
View more Courses
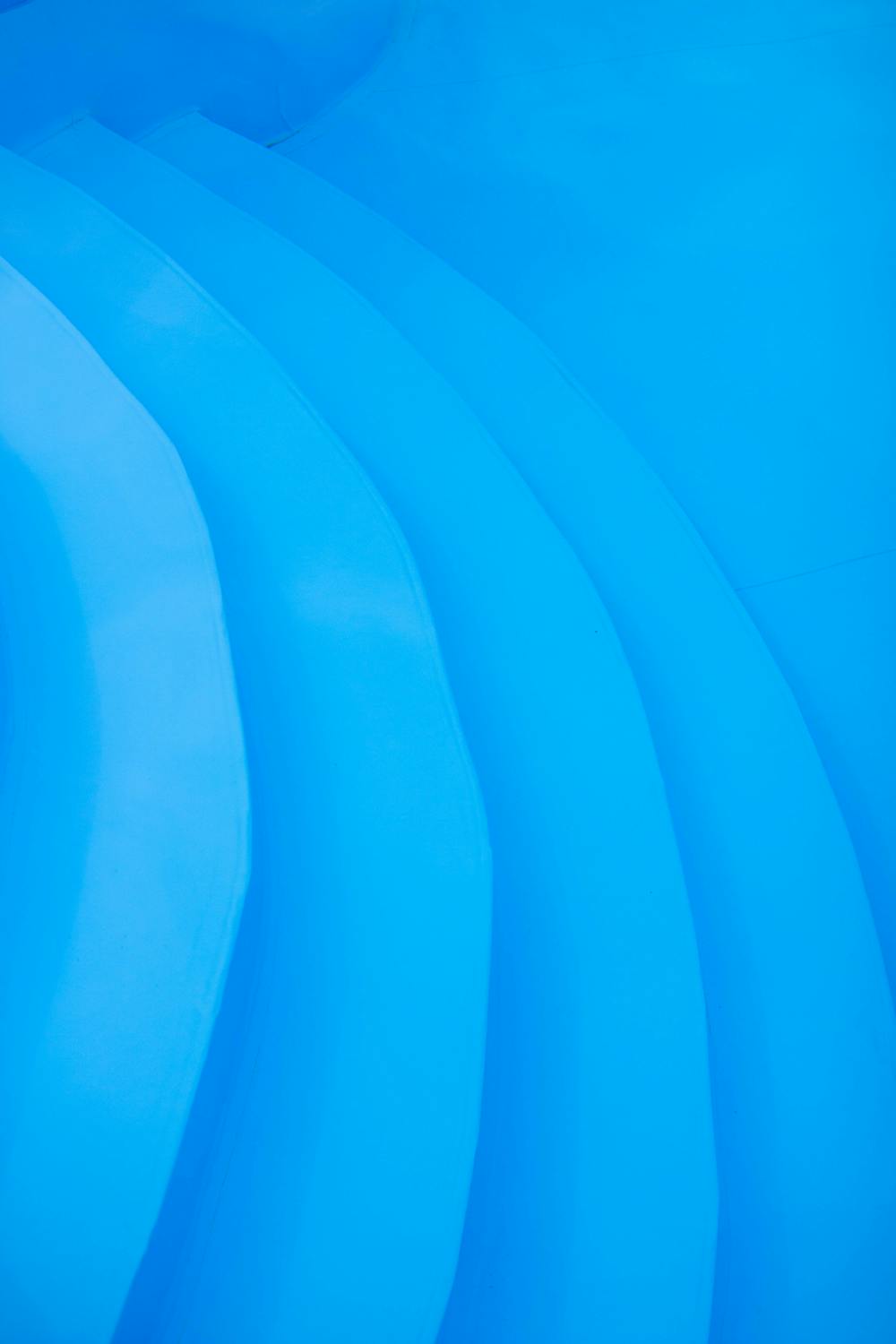