Omdena Academy Courses

Machine Learning for Smart Health Systems
November 29, 2024

For whom is this course?
This course is for anyone interested in applying machine learning for disease detection, monitoring, and other biomedical applications.
Objective
- Learn how to process physiological signals e.g. ECG, EEG, PPG, respiration captured from the human body
- Feature extraction from physiological signals
- Feature selection for the detection of specific diseases of interest
- Importance of confounding factor analysis in biomedical applications
- Disease classification and severity estimation using machine learning and deep learning models
What will you learn?
- Technical skills are essential, but not enough, non-technical and domain fields of studies are still essential if you want to understand data science vs its application.
- Current and future global challenges in the sector
- How data science or artificial intelligence would be applied.
- Data science and the necessities to keep learning for life.
- Instructor-led online course with guided labs
- Real-world, practical assignment(s) leading to project
- Application in biomedical and health systems
Prerequisites
- Basic Python
Syllabus
Week | Instruction (1 hr) |
Lab (guided + unguided) 1+ 3 hrs |
Week 1 (5 hrs) |
Intro to smart health, and physiological signals. ETL and visualization in smart health |
Basic signal processing-loading, denoising ECG, acceleration, PPG signal and detecting biomarkers |
Week 2 (5 hrs) |
Feature extraction, Feature selection in machine learning, and co-variate analysis | Extract features from ECG and PPG, acceleration signals, Feature selection for sleep apnea & heart disease classification, and gait analysis |
Week 3 (5 hrs) |
Developing machine learning & deep learning models for disease classification | Sleep apnea and heart disease classification using ensemble and deep neural networks |
Week 4 (5 hrs) |
Deploying machine learning & deep learning models for disease classification | Export portable and deployable model using WEKA & TF-Lite, cloud computing |
Week 5 (10 hrs) |
Case study guidance & evaluation | Real-world case study (10 hrs) |
Instructors
Course Info
Certificateyes
Duration30 hours
Start DateNovember 1, 2021
Last Registration DateN/A
No of Students35-40
Skill Levelbeginner
View more Courses
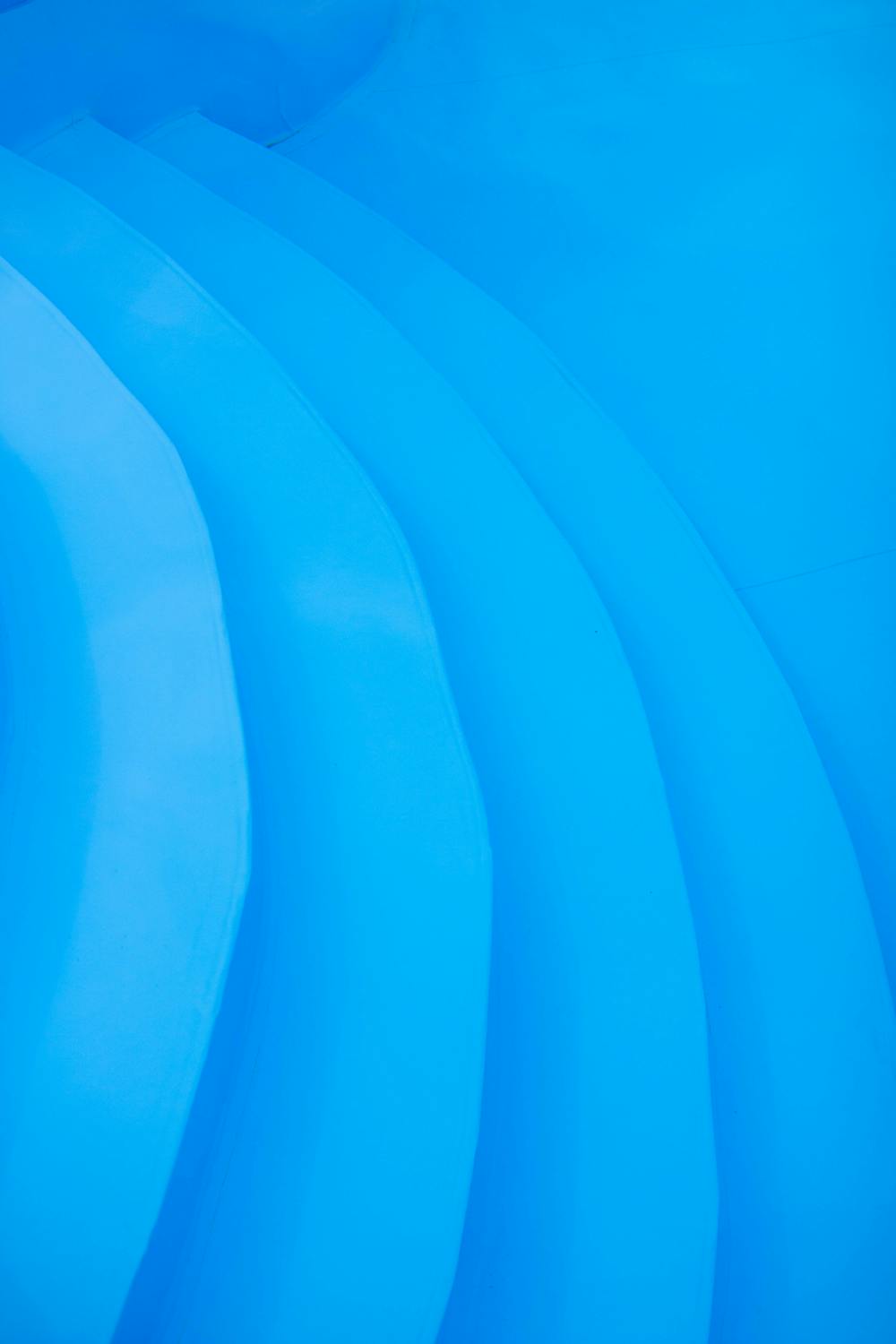
View all courses from Omdena Academy Go Back