Detecting Wildfires Using CNN Model with 95% Accuracy
April 28, 2020

The Problem: Wildfires and a Convolutional Neural Network
2019 was marked by very big fires. Not only the Notredame cathedral in Paris, and the National Museum in my country Brazil but entire complex ecosystems like the Amazon forest Wildfires and more recently in Australia. Before we dive into our finished product of, how to detect and stop wildfires early on with our community-build AI tool, let us understand how forest fires start.
- Natural fires: Generally, natural fires are started by lightning, with a small portion originated by spontaneous combustion.
- Human-caused fires: Humans cause fires in multiple ways such as smoking, recreation, soil preparation for agriculture, and so on. Man-caused fires represent the greatest percentual share of fires, but natural-caused fires represent larger burned land areas. This happens because the man-caused are detected earlier, while natural fires can take hours to be identified by the competent authorities.
Regardless of the causes, when a forest like in the Amazon starts to burn, the fire can spread and reach speeds of up to 23 km/h and reach temperatures of 800 °C (1470 °F) destroying plant and animal life within a few hours (sometimes even contributing to species extinction)
Even worse, fires damage the planet through CO2 that will contribute to global warming.
In addition to disrupting the climate, it impacts the sky and the quality of the air of a huge metropolitan city like São Paulo, the most important economical and productive center for my country.
At 3 pm, August 19th, 2019, a black sky appeared as a result of the meeting of a cold front with the fire particulates stemming from the Amazon and midwest fires in my country.
The day became night, and the feeling was that we were living in a biblical plague as described in the Old Testament. Really scary!

Pictures of São Paulo’s sky at 3 pm on August 19th, 2019
Among much misinformation, one post from NASA stood out by shedding the fundamental light of science on the matter.
In the image below, you see a colored high-resolution satellite image showing how the fire smokes spread to the southeast states of my country.
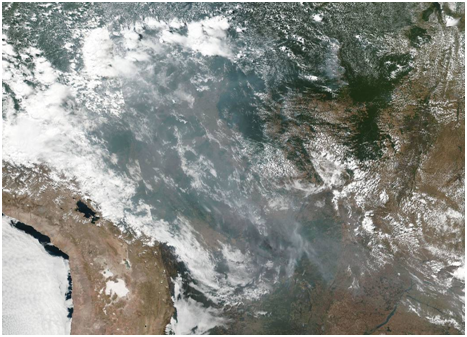
By NOAA/NASA’s Suomi NPP using the VIIRS (Visible Infrared Imaging Radiometer Suite) on August 20th, 2019
The solution
Sintecsys´s growing customer base of clients on farms and forests can confirm. The company installs cameras on top of communication towers to capture images that are sent to a monitoring center. Once there is fire (or smoke) detected on images, it sends alerts and fire fighting actions. This saves lives and infrastructure costs.
Sintecsys is not alone in its mission as there are many other companies around the world dedicated to this mission also in a very successful way.
The company installed 50 towers distributed in Brazil (2019 data.
To extend the customer reach and scale their business model to thousands of cameras with the capability of accurately and quickly detecting wildfire outbreaks, Omdena’s AI capabilities come into play.
Omdena is a global platform where organizations collaborate with a diverse AI community to build solutions for real problems in a faster and more effective way.
How the team solved the problem
#1 Scoping the problem
To tackle this problem, Omdena and Sintecsys agreed to deal with day images in their first joint challenge and in a second challenge improve the solution by dealing with night images.
The main difference between day and night images for fire detection is that during the day images usually show smoke and during the night these images show live fire. Both sunset and dawn, where smoke and live fire coexist on images, represent boundary conditions for the problem.
#2 Working on the dataset
The dataset was really big comprising footage and images from different cameras with and without fires outbreaks happening. Combining the original images given, our team had almost 7.600 images of 1920 x 1080 size (day images without fires outbreaks, day images with fires, and some night images (around 16%)) to start labeling.

Samples from the datasets
To add even more images, Gary Diana built an algorithm to successfully extract images from the footage and at the same time avoiding the generation of images with the same landscape among them (de-duplication). This initiative added another 1.150 images of 1280 x 720 size to our dataset.
#3 Labeling with Labelbox
Having the datasets prepared for labeling, we gathered around 20 people dedicated to the task, created the environments on Labelbox, which is the best tool available for computer vision by allowing labeling data, managing its quality and operating a production training data pipeline, and then, at last, we started to make tests and to label the final datasets.
I managed the task but I received huge support from Alyona Galyeva who helped the whole team not only by labeling but also by reviewing and managing everyone´s work.
In her own words:
It always starts with a mess when a group of people collaborates on a labeling project. In our case, Labelbox saved us a lot of time and effort by not allowing multiple users to label the same data. On top of that, it made our lives easier by proposing 4 roles: Labeler, Reviewer, Team Manager, and Admin. So, nobody was able to mess with data sources, data formats, and, of course, the labels made by other people.

Labelbox interface for labeling, managing and reviewing labels
Having both datasets labeled, the next train, validation, and test files were generated by the data pipeline team.
#4 Building the models
From the start, the team searched and studied several top-notch papers with different techniques that could be applied to solving the problem.
The challenge team created several teams in different tasks, each one focused on trying different approaches: mobile net, semantic segmentation, Convolutional Neural Networks (CNNs) — from simple architectures to more sophisticated ones.
Another great testimony of this step comes from Danielle Paes Barretto:
It was inspiring to see people eager to achieve great results. I tried to help in all tasks; from labeling the data to building CNN models and testing them on our dataset. We also had frequent discussions which in my opinion is one of the greatest ways of learning. All in all, it was an amazing opportunity to learn and to use my knowledge for the good while meeting great people!
In addition, different techniques were successfully applied to improve results like creating patches of different sizes on original images and training over patches, data augmentation (e.g. horizontal and vertical flipping), denoising images, etc.
#5 Results
The final solutions were able to reach a recall between 95% and 97% while having a false positive rate between 20% and 33%, which means that these solutions were extremely successful in catching 95% to 97% of the real fires outbreaks. While the challenge partner Sintecsys is extremely happy with the results, in our second challenge, we will improve the current models by adding night time images.
You might also like
Want to work with us too?



